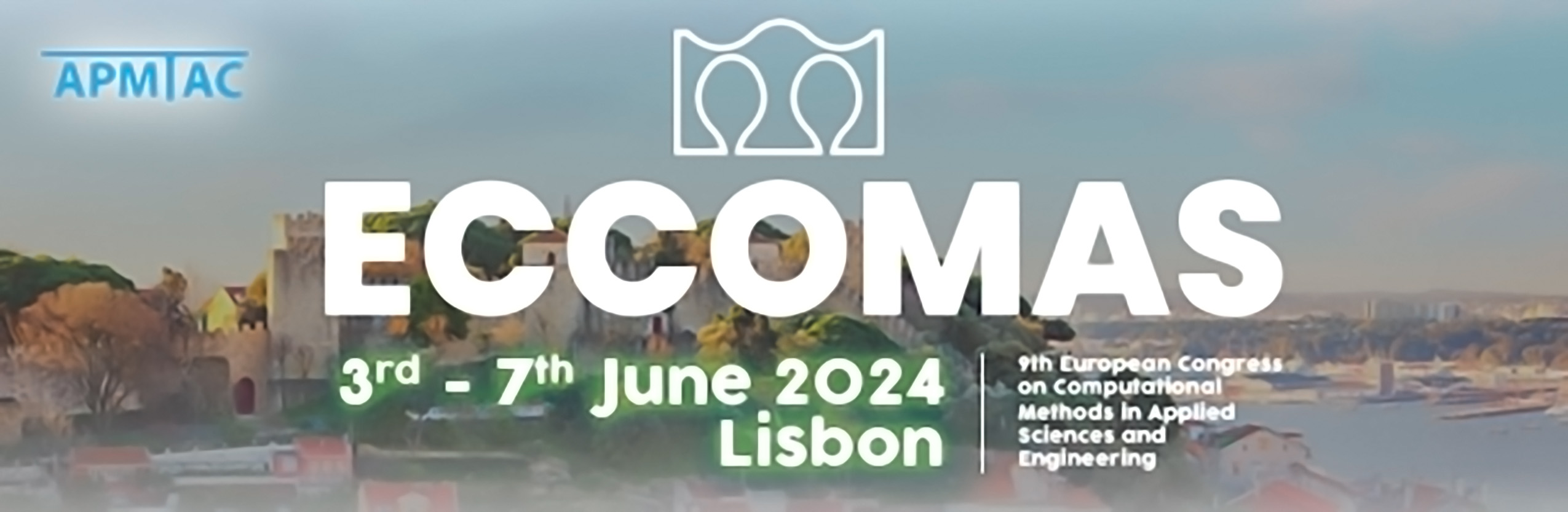
MS109A Combining Physics-Based and Data-Driven Approaches for Uncertainty Quantification I
MS Corresponding Organizer: Dr. Augustin Persoons (KU Leuven)Scheduled presentations:
-
Advancements in Hybrid Modeling for Battery Electrode Manufacturing Utilizing a Physics-inspired Data-driven Approach
S. Hosseinhashemi*, C. Thon, C. Schilde -
A Practical Data Generation Framework for 4D Automotive MIMO Radar: Enabling Deep Learning and Radar Performance Analysis
M. Jürgensen*, M. Vossiek, J. Fuentes Michel -
A Point-Cloud Enhanced Transfer Learning Approach for Crashworthiness Analysis
G. Colella*, V. Lange, F. Duddeck -
Mapping Manufacturing Variability to Composite Pressure Vessels’ Geometry
R. Santos*, A. Persoons, D. Moens -
Machine Learning Prediction of Random Property Fields From Microstructure Images
P. Gavallas*, J. Bourinet, C. Mattrand, G. Stefanou, D. Savvas -
Model Uncertainty Quantification and Selection for Deep Learning-based Simulation of Hysteresis with Stiffness and Strength Degradations
J. Jeon*, J. Song, O. Kwon