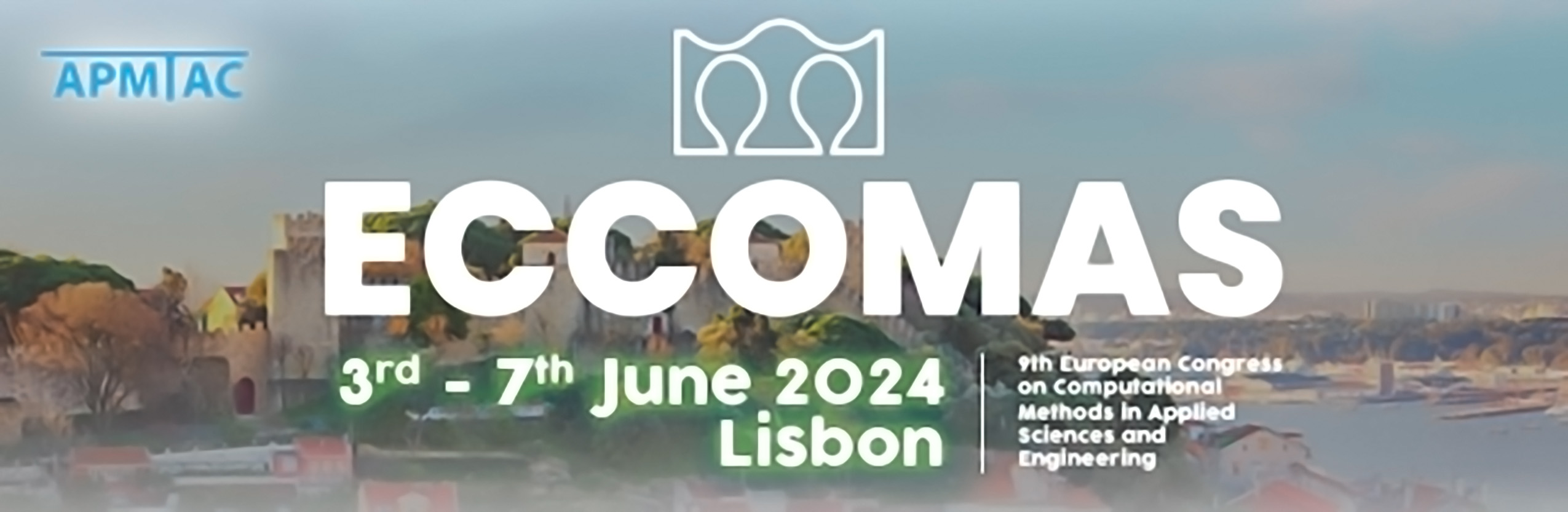
A Practical Data Generation Framework for 4D Automotive MIMO Radar: Enabling Deep Learning and Radar Performance Analysis
Please login to view abstract download link
The application of machine learning in automotive radar systems presents severe challenges. In particular, the availability of raw radar data tailored to specific radar configurations and annotated datasets is limited. In this paper, we propose a novel method to reduce the lack of available datasets and bridge the gap between recorded datasets (which fit one type of radar) and sophisticated ray tracing simulations (which are computationally expensive and based on assumptions). Our approach utilizes recorded features from existing radar systems currently used in production. We specifically accumulate radar detections (as defined in ISO 23150:2023) over multiple cycles to increase the resolution of a given radar and minimize fluctuation in the features obtained. To achieve more representative results, we include clutter in the simulation. The accumulated features, which we will refer to as pseudo-scatter points, are then treated like scatter centres to calculate raw spectra for virtual radar systems with arbitrary antenna arrangements. The following processing steps extract range, velocity and angular information. We compare simulated radar data from a digital twin with measured radar data in an identical scenario to validate our method. Our framework provides a practical and versatile solution for radar data generation, facilitating the development of deep neural networks and the predevelopment of radar systems. Although it may not achieve the level of fidelity provided by ray tracing simulations, we demonstrate that our approach offers a cost-effective method for generating high-quality radar data for advanced driver assistance systems (ADAS) applications.