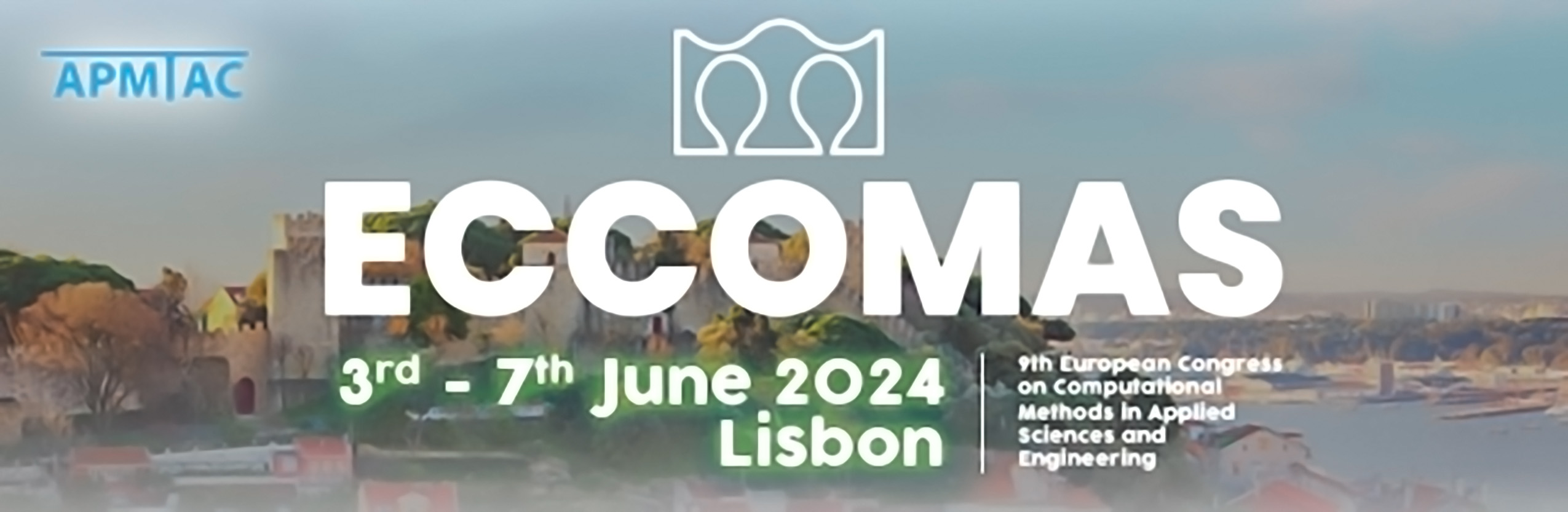
Advancements in Hybrid Modeling for Battery Electrode Manufacturing Utilizing a Physics-inspired Data-driven Approach
Please login to view abstract download link
Advancements in Hybrid Modeling for Battery Electrode Manufacturing Utilizing a Physics-inspired Data-driven Approach Somayeh Hosseinhashemi, Christoph Thon and Carsten Schilde ¹ 1 Institute for Particle Technology (iPAT), Technische Universität Braunschweig, Volkmaroder Str. 5, 38104 Braunschweig, Germany s.hosseinhashemi@tu-braunschweig.de, c.thon@tu-braunschweig.de, c.schilde@tu-braunschweig.de Key Words: Hybrid Modeling, Battery Manufacturing, Genetic Algorithms, Neural Networks, Symbolic Regression, Symbolic Transformer, Physics-Inspired Data Analysis The pursuit of optimized industrial processes is increasingly turning towards intelligent systems that minimize trial-and-error methodologies. In battery electrode manufacturing, this work presents significant advancements in a hybrid model that synergizes physics-inspired knowledge with data-driven artificial intelligence techniques. Building upon our previous work, which established a foundational hybrid model using genetic algorithms for symbolic regression, we have improved our approach by using the symbolic transformer concept to address the complexities of battery electrode production. We have developed an symbolic transfer model that interfaces with a genetic algorithm to uncover and quantify the intricate physical relationships in data. This updated model extends its predecessor by incorporating new insights into the physical properties of materials, enabling better interpretation of parameters and their impact on the production process. The centerpiece of our method is the determination of symbolic expression for an unknown function that governs the relationship between process parameters and system behaviors. Our approach utilizes a data table format {x1, ..., xn, y} to streamline the analysis, where y = f (x1, ..., xn). The culmination of this research is a robust white-box model that not only predicts but also informs the optimization strategies for battery electrode production. This presentation will illustrate the iterative development of our model, underscored by a case study demonstrating improved battery performance through the application of our hybrid modeling technique. The implications of this work are significant, offering a promising avenue for accelerating innovation and efficiency in battery technology through intelligent, physics-informed process modeling. REFERENCES [1] S.Hosseinhashemi, Y.Zhang, C. Thon and C. Schilde. Process insights with physics-inspired data-driven