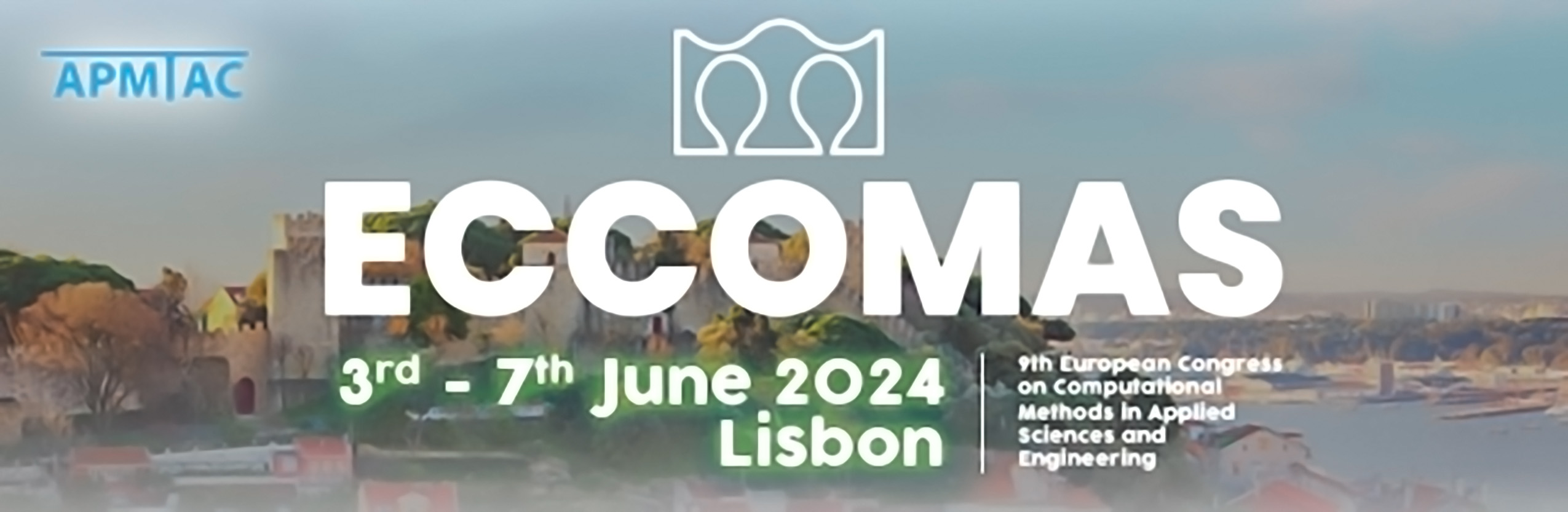
Machine Learning Prediction of Random Property Fields From Microstructure Images
Please login to view abstract download link
The standard method of computing equivalent properties via finite element (FE) based homogenization [1] can be computationally expensive, especially in the case of complex microstructures. In this work, an efficient alternative based on neural networks is proposed for predicting random property fields of composite materials from images of their microstructure. More specifically, the FE homogenization is substituted by a convolutional neural network (CNN) which takes as input a microstructure image and returns the equivalent properties. According to [2], in order to compute a random property field from an initial image, windows or stochastic volume elements (SVEs) are extracted using a moving window technique and homogenization is applied to each SVE obtained. By replacing FE homogenization with a CNN [3], the proposed approach can greatly accelerate the random field computation process. The CNN is trained on randomly generated microstructures with a volume fraction varying from 0 to 45%, which are representative of the aforementioned SVEs. With the adopted CNN architecture, the edge pixels of the composite image are used as additional input parameters, and their effect on the equivalent properties is highlighted. The proposed method is compared with standard FE homogenization on simulated and real composite images and is found capable of predicting random fields with acceptable accuracy at a fraction of the computational cost. ACKNOWLEDGEMENTS The GREYDIENT project leading to this application has received funding from the European Union’s Horizon 2020 research and innovation program under the Marie Sklodowska-Curie grant agreement No 955393. REFERENCES [1] C. Miehe, A. Koch, Computational micro-to-macro transitions of discretized microstructures undergoing small strains, Archive of Applied Mechanics 72, 300-317 (2002). [2] D. Savvas, G. Stefanou, M. Papadrakakis, Determination of RVE size for random composites with local volume fraction variation, Computer Methods in Applied Mechanics and Engineering 305, 340-358 (2016). [3] F. Aldakheel, E.S. Elsayed, T.I. Zohdi, P. Wriggers, Efficient multiscale modeling of heterogeneous materials using deep neural networks. Computational Mechanics 72, 155-171 (2023).