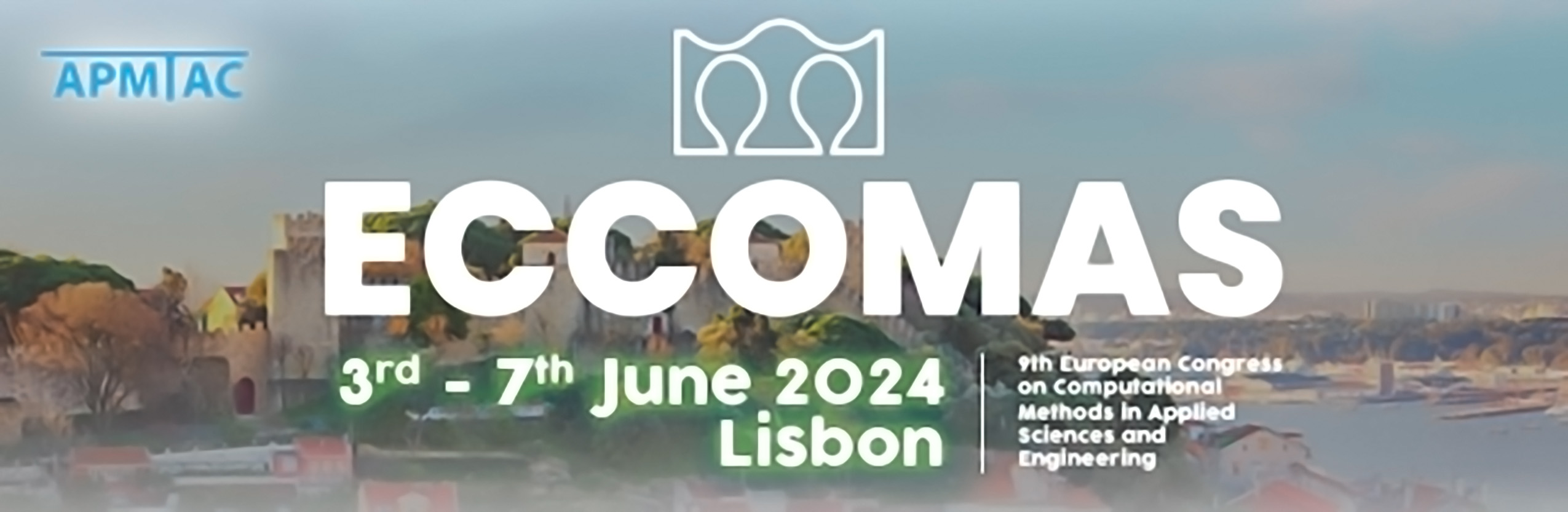
Combination of FEM and machine learning models for enhanced dam safety assessment
Please login to view abstract download link
Safety assessment of dams is primarily based on the comparison between the observed behaviour –as recorded by the monitoring devices– and the expected response in front of the acting loads. The latter is computed by some predictive model, often based on the finite element method (FEM). Such models can account for the physical phenomena involved, but their accuracy is limited: the application of complex constitutive laws requires accurate estimation of material parameters, which is often difficult to achieve; the same applies to the joint elements or the thermal boundary conditions. In recent years, machine learning models are increasingly used for this purpose and have shown to be more accurate, taking advantage of the monitoring data available [1,2]. However, data-based models also present serious limitations: they are not reliable to predict behaviour in extraordinary situations and are not applicable when time series are short or of low quality. We explored the possibilities of the combination of data-based and physics-based models to accurately predict dam behaviour both in ordinary and extreme load scenarios, as well as in a variety of settings regarding the quality and quantity of monitoring data. The proposed approach is based on applying a relatively simple FEM model, easy to create, with low computational cost and which can be generated without detailed information on the environmental variables or the material properties. The predictions of the FEM are later corrected using a model based on machine learning. The effectiveness of this approach was evaluated on a real case study of a double-curvature arch dam, based on the time series of monitoring records and a variety of hypothetical scenarios representing realistic situations.