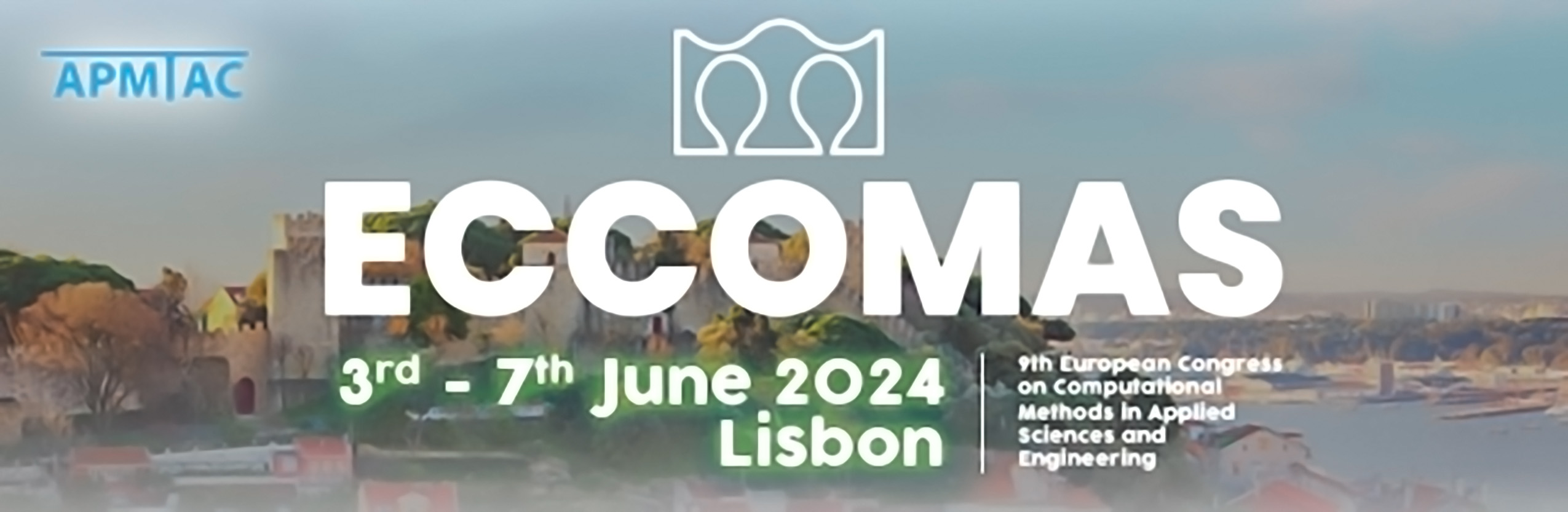
Identifying active subspaces in molecular dynamics using machine learning
Please login to view abstract download link
The deep active subspace method is a neural-network based tool for the propagation of uncertainty through computational models with high-dimensional input spaces. Unlike the original active subspace method, it does not require access to the gradient of the model. It relies on an orthogonal projection matrix constructed with Gram-Schmidt orthogonalization, which is incorporated as a linear encoder into a neural network, and optimized using back propagation to identify the latent variables, i.e. the active subspace of the input. We assess the performance of the deep active subspace method on classical molecular dynamics with a particular focus on uncertainties in the high-dimensional force-field parameters, which affect key quantities of interest, including macroscopic material properties and binding free energy predictions in drug discovery and personalized medicine.