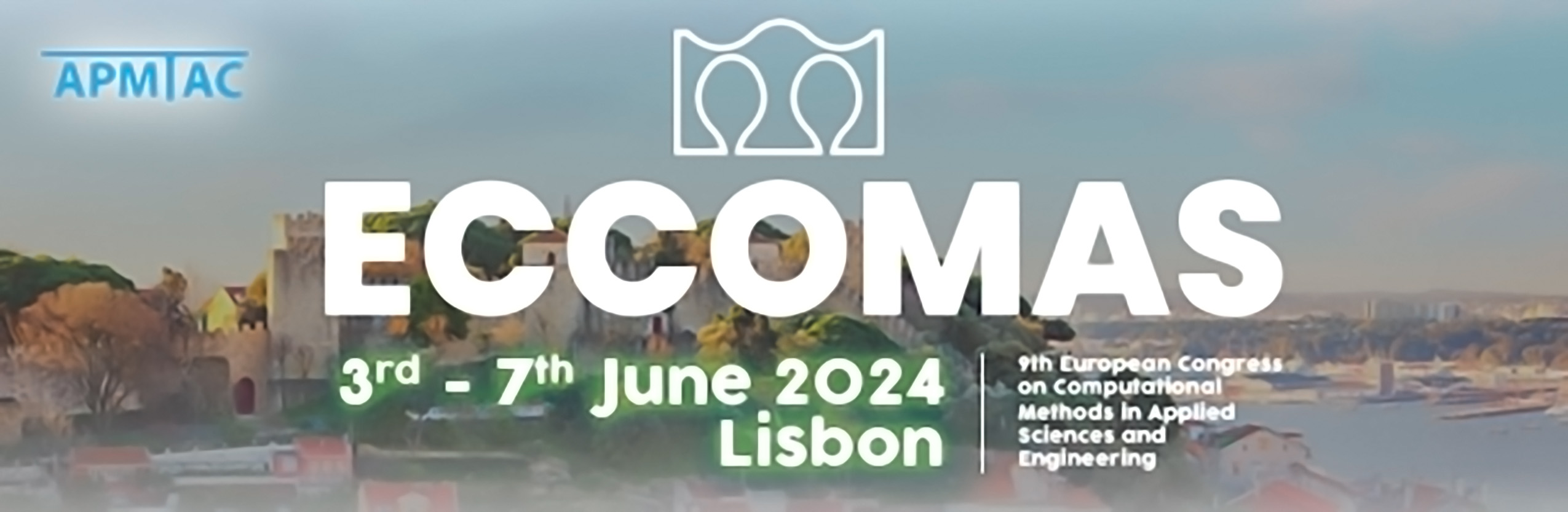
A-priori Energy-Biased Training for the Prediction of Closure Terms of the VMS Navier-Stokes Equations
Please login to view abstract download link
The authors present an energy-biased training approach for predicting the closure terms of the Navier-Stokes equations discretised in a Variational Multiscale framework. The approach initially involves formulating a constrained objective function, which is transformed into an unconstrained problem suitable for neural network training using the Augmented Lagrangian method, inspired by the technique in [Nocedal&Wright2006]. The constraints force the predicted closure terms' energy contribution to be smaller than the truth, regardless of whether the energy contribution is backscatter-related or dissipation-related. Effectively, the approach controls energy contributions through a-priori measures rather than a-posteriori measures. The approach was applied to obtain the H1 projection of a Re_tau = 180 turbulent channel flow on a 32³ mesh. Eight MLPs were trained to predict the closure terms associated with the weighting functions of each linear hexahedral element. Each network used 217 features, and five hidden layers with 600 neurons each. The final data-to-parameter ratio was ~19.6 : 1 (30 932 992 : 1 579 811) per neural network. A-priori evaluation of the networks' outputs demonstrated a tendency to predict closure terms yielding the desired energy dissipative behaviour. This was true for both closure terms that yield energy gain and energy loss via backscatter and cascade, respectively. In spite of the energy bias, the correlations of the closure term predictions with their truth values were demonstrated to be greater than 0.85 for all positions between the channel walls.