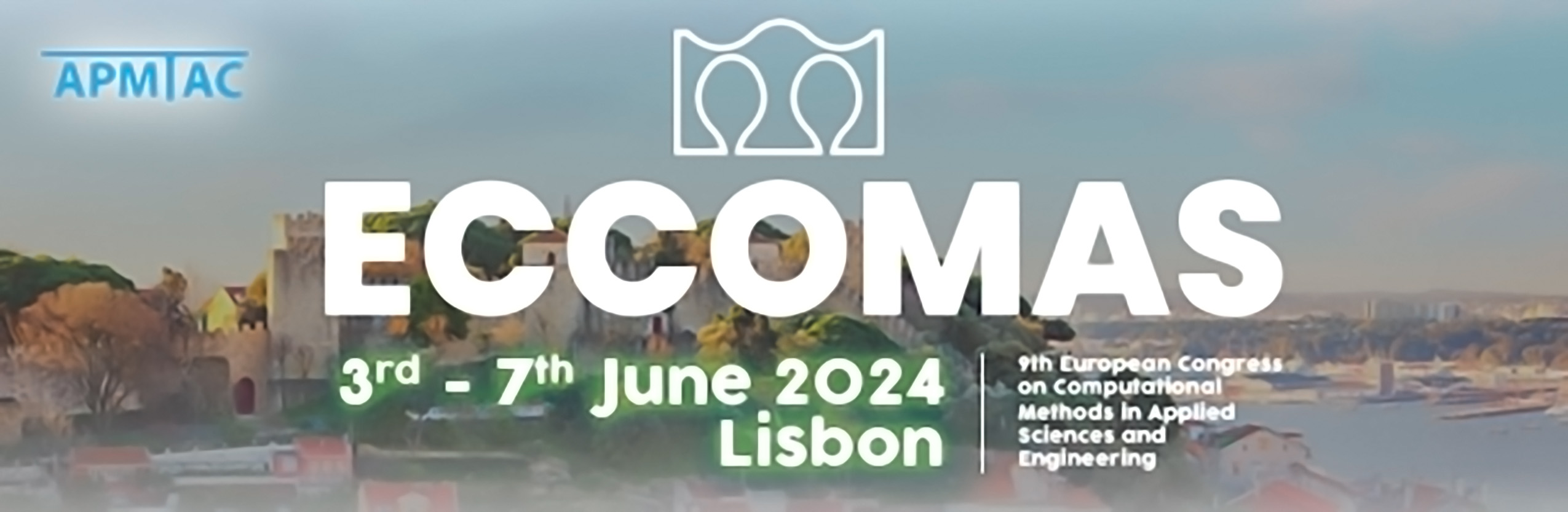
Active learning of constitutive surrogate models from molecular dynamics simulations
Please login to view abstract download link
Material properties, particularly mechanical ones, are intrinsically multiscale, emerging from the material's structure ranging from the atomic to the continuum structure. Multiscale modelling and simulation based on the assumption of a separation of scales in the architectures of nanomaterials appears as a promising alternative to trial-and-error experiments for material design. The current standard for multiscale simulation is hierarchical, information is passed bottom-up, from one scale to another, in a sequential fashion. However, this may not be sufficient at times, two or more scales need to be captured simultaneously as they influence each other synergetically. This is typically the case when the nanoscopic structure of the material evolves with loading applied on much larger scales. A wide range of applications are concerned, non-exhaustively: from the emergence of mechanical properties in polymers showing a mixture of amorphous and crystalline regions at the microscopic scale; ion conductivity in mechanically-loaded electrolytes; chemical attack and corrosion in indented materials. To tackle such challenges, we designed a multiscale computational method coupling concurrently many molecular dynamics simulations to one continuum finite element simulation, enabling the prediction of realistic mechanical observables with chemical specificity. However, the technique is prohibitively expensive even for the largest supercomputers. Nonetheless, by integrating recent machine learning developments, in particular clustering and surrogate modelling, we have improved the tractability of such a technique. In this talk, we will present our most recent developments in terms of multiscale simulation and applications to resolve pressing questions in materials science.