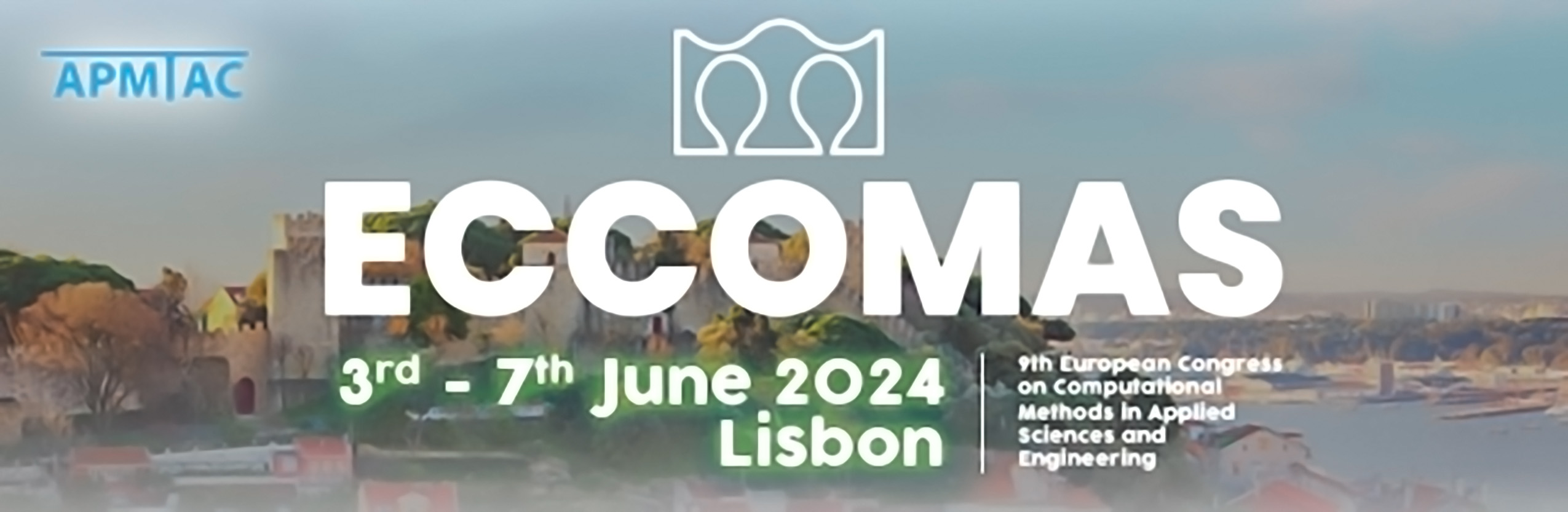
Swarm Intelligence Based Calibration of Discrete Element Models for Masonry Structures
Please login to view abstract download link
The presentation addresses the calibration of constitutive parameters in discrete element models of masonry structures. The goal is to provide automatic methods that can closely align the outcomes of computer-simulated loading procedures with real physical experiments on masonry structures, by properly choosing the material characteristics in the simulated reality. The Discrete Element Method (DEM) represents a masonry structure as a collection of solid bodies either rigid or deformable. These bodies interact through various types of contact forces such as compression, friction and other interactions such as tension or cohesion. DEM can easily simulate phenomena leading to structural failure, such as frictional sliding and joint separation, assuming accurate modeling of mechanical behavior of both the individual bodies and their contacts. Manual calibration, which has been the usual method until now, is time-consuming. It requires the tuning of micromechanical parameters (such as contact stiffnesses, friction angle or dilation) to match the simulated results with experimental data. The key message of the presentation is that automating this essential step with artificial intelligence can significantly accelerate, ease and streamline the process. The presentation recommends various swarm intelligence algorithms, recently tested and compared by the authors, for modeling a masonry arch. The load-displacement behavior of the arch had been analyzed in laboratory measurements [1] and then simulated with the discrete element software 3DEC [2] in the past. We tested the Trust-Based Particle Swarm Optimisation, Marine Predator Algorithm, and Golden Eagle Algorithm [3], [4]. A novel two-step approach is also introduced, enhancing the performance of these optimisation algorithms. Furthermore, given the non-uniformity of material properties in masonry structures, the presentation also includes the calibration of the random normal distribution of these properties, focusing on their mean and standard deviation. This addresses the challenge of varying material characteristics across the structure. Additionally, the presentation introduces a methodology using Convolutional Neural Networks to precisely capture the failure mode of the structure.