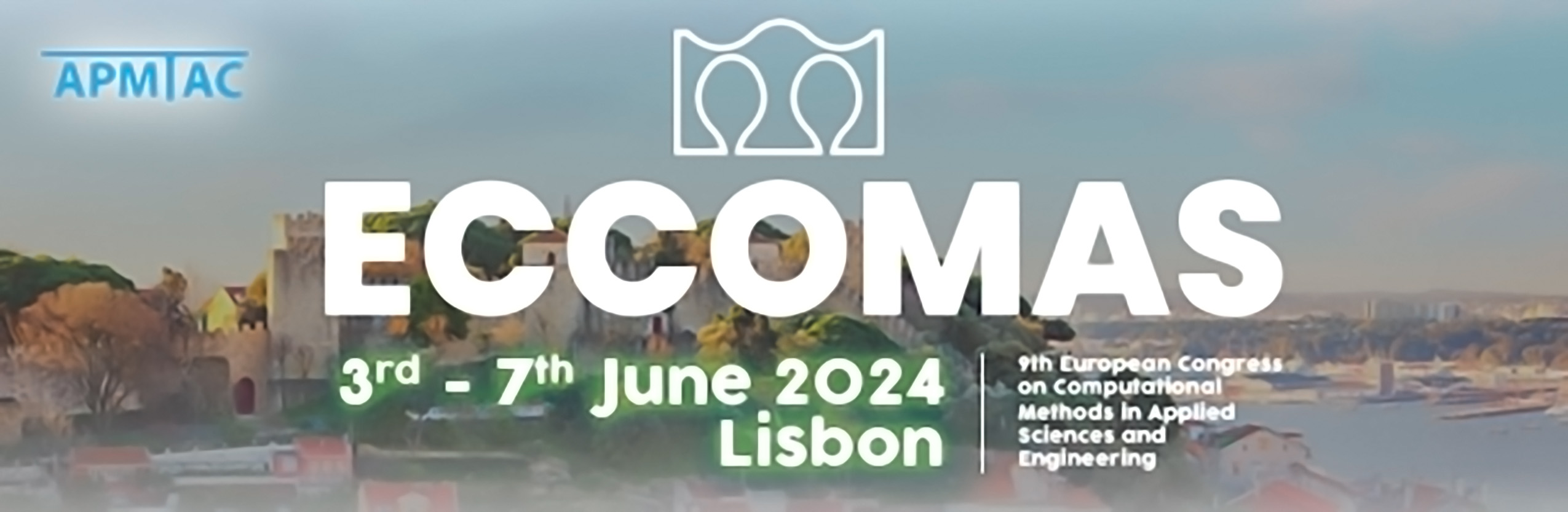
Unsupervised Data-driven Tool for Track Support Conditions Assessment – First Results
Please login to view abstract download link
Taking advantages of recent developments in machine learning procedures, railway researchers have been creating new tools and methodologies to improve the structural health monitoring solutions that were developed over the last century [1]. These tools are now used to assess a wide range of railway related structures and cases [1]. However, the current vehicle-based railway monitoring methodologies cannot usually provide reliable data on track subgrade conditions [2]. This is a key issue since subgrade condition significantly influences track dynamic response and overall track support conditions [2]. An alternative and novel methodology to assess railway track support conditions is now under development and validation, which is based on modal analysis of the characteristic frequencies of the multi-element system composed by an instrumented railway vehicle and the railway infrastructure under assessment. Furthermore, an unsupervised data-driven procedure is currently being developed to improve the capabilities of the proposed track monitoring methodology on feature extraction from collected data. This tool is also expected to improve the overall reliability of the proposed methodology. To ensure the intended continuous assessment of the railway track, this procedure is formed by a sequential combination of autoregressive models, principal components analysis and clustering algorithms, in a sliding window process over the collected input data. This paper provides an overall description of this procedure and the obtained preliminary results, which are based on numeric simulations performed using the Simpack® software.