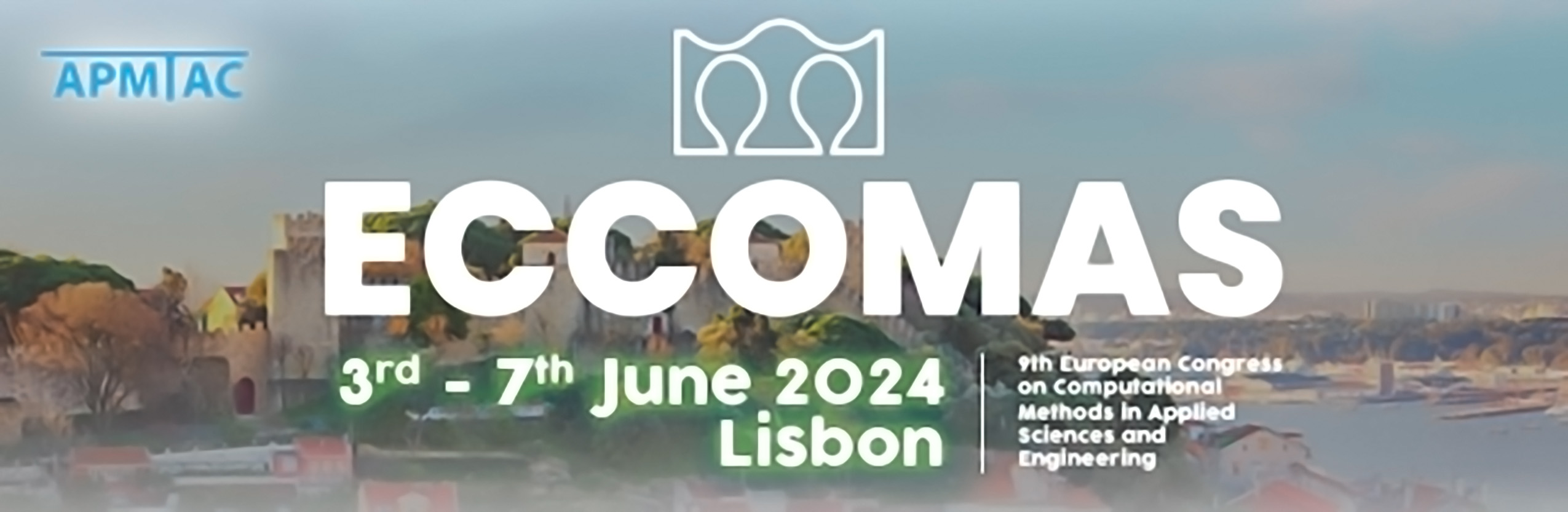
AI Model to Predict in-situ Relative Permeability of Rock Formation
Please login to view abstract download link
The study of multiphase flow behaviour in porous media holds critical significance for numerous key industrial processes, such as oil and gas development, geothermal energy extraction, groundwater pollution mitigation, and nuclear waste deposition. Among the various material properties influencing porous media flow, permeability stands out as arguably the most pivotal. While the industry conventionally relies on lab-based experiments to measure permeability, this approach suffers from drawbacks like high costs, limited sample availability, and poor repeatability. Recent research has displayed promising advancements in simulating and predicting absolute permeability. However, there has been less success in predicting relative permeability, despite its heightened sensitivity and profound impact on the underlying multiphase flow processes. This paper concentrates on predicting relative permeability, specifically considering the influence of in-situ stress conditions. A novel AI-based microstructure reconstruction method has been devised and applied to generate a comprehensive array of digital rock models that faithfully replicate real-world formation samples. Subsequently, a robust mapping scheme has been developed to convert these digital rock models into spherical DEM (Discrete Element Method) models, facilitating precise stress analysis. The simulated stress and deformation data are then transposed onto the digital rock models. This integration allows for the consideration of in-situ stress conditions in porous media flow simulations using the lattice Boltzmann method. Following DEM and LBM simulations across the complete digital rock sample set, machine learning techniques are employed to create a symbolic equation predicting relative permeability using image-based statistical descriptors. The result is an immediately applicable tool enabling rapid estimation of relative permeability under in-situ stress conditions, all derived from a CT rock scan. This work amalgamates various modelling, data, and AI techniques to offer a pragmatic solution to the long-standing challenge of relative permeability prediction, which has stymied the industry for an extended period.