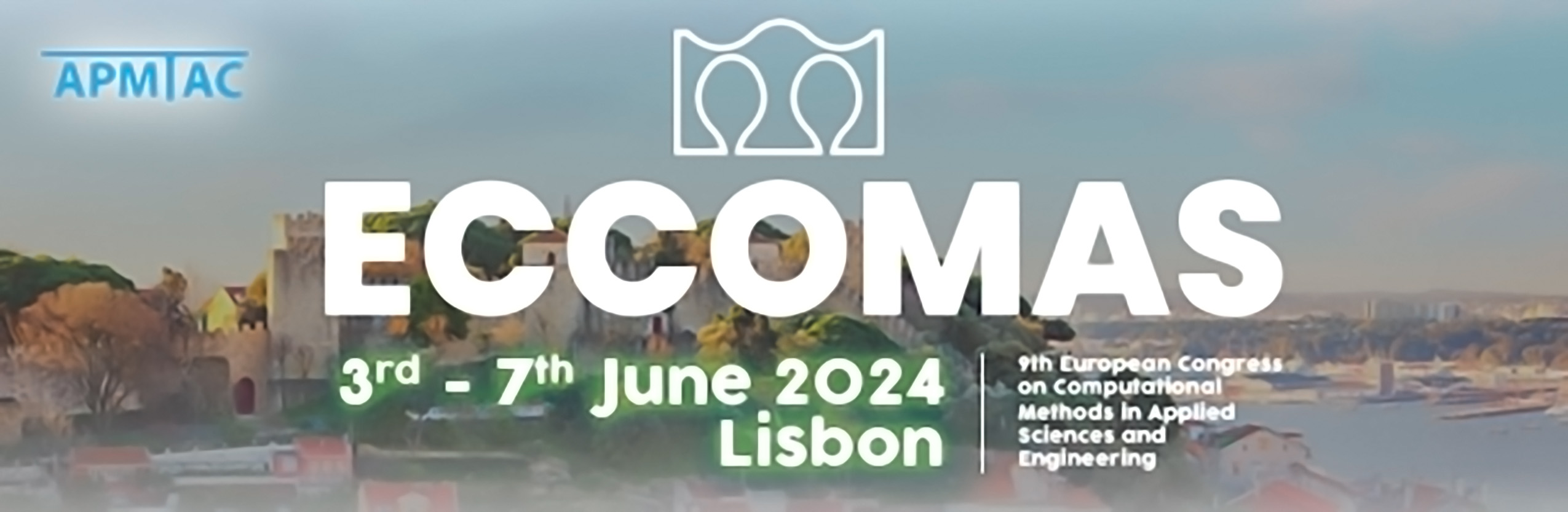
Accelerating Multiscale Modeling of Delamination With a Suite of Surrogate Models
Please login to view abstract download link
Fiber-reinforced composite laminates are intrinsically multiscale materials: at the microscale, individual fibers and surrounding resin can be discerned, whereas the mesoscale is composed of a combination of plies that form a laminate. Leveraging this multiscale nature is beneficial when building high-fidelity models of composite behavior, as failure phenomena of interest are typically inherent to the mesoscale but material behavior can be more accurately described at the microscale. More specifically, we are concerned with modeling crack propagation at the mesoscale while keeping track of the micromechanical evolution of plasticity and distributed cracking ahead of the crack tip. This requires a concurrent multiscale approach (FE2) involving the embedding of microscopic FE models at each and every mesoscopic Gauss point. However, the computational cost associated with this type of modeling is enormous, creating the need for efficient and accurate ways of accelerating the involved microscale computations. The solution we present in this work involves combining two different surrogates in a single framework. We model delamination crack growth in mixed-mode bending (MMB) tests at the mesoscale while including matrix plasticity, matrix damage and fiber-matrix debonding at the microscale. For crack growth we employ a Gaussian Process-based active learning framework in which a GP model is conditioned on observations of cohesive tractions and displacement jumps coming from a small number of micromodel computations. The Bayesian nature of the employed GP models is exploited by using the resulting predictive variance to drive active learning. For the surrounding bulk material we employ a hybrid surrogate model combining a neural network-based encoder-decoder architecture with a set of fictitious material points embedded in the latent space of the network that convert encoded local strains to local stresses using the exact same physics-based constitutive models employed at the microscale. The state variables of the fictitious points can be seen as a low-dimensional latent representation of the state of the micromodel and allows for path-dependent material behavior to be captured naturally. The combined framework allows us to perform FE2 simulations that would otherwise be out of reach, and help elucidate energy dissipation mechanisms due to delamination.