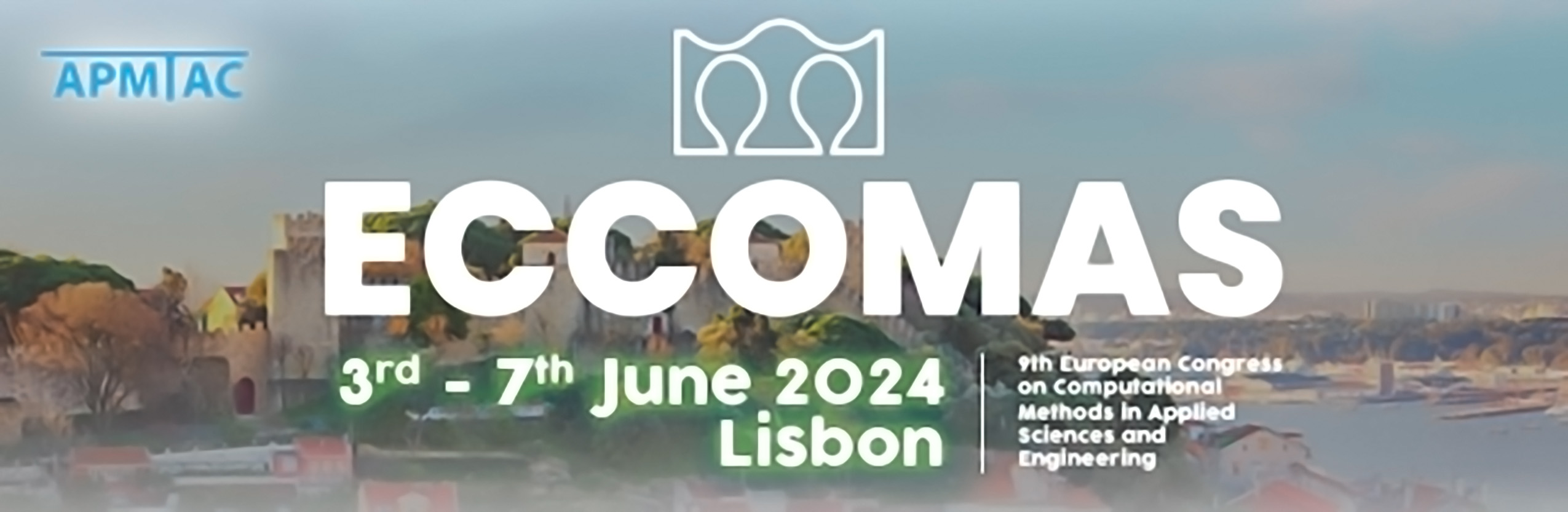
Prediction of temperature-dependent thermo-mechanical properties of PCB conductive layers using convolutional neural network
Please login to view abstract download link
Deriving the composite properties of printed circuit board (PCB) conductive layers is a particular challenge due to the intricate patterns of conductive artwork. While the use of semi-empirical methods, i.e. Rule-of-Mixture methods and such, struggle to determine the true orthotropic material behaviour in this case; the use of solely Finite Element (FE) simulations poses another challenge: creating a unique model for each structure of interest is highly time-consuming and contradicts time-to-market objectives in the industry. This together prompts development of the novel modeling approaches, including those involving machine learning. In foregoing work the authors presented prediction of orthotropic thermo-mechanical properties of patterned copper conductive layers using the convolutional neural network (CNN) in the way that the necessary level of detail for PCB modeling was ensured [1]. However, for most PCB reliability analyses, such as board warpage prediction, the derivation of temperature-dependent material properties is crucial. Hence, building upon our previous research, we have extended our model to address temperature dependency with the further validation of the results. The trained network was able to make highly accurate predictions of the homogenized temperature-dependent thermo-mechanical properties for unseen copper-resin patterns. Unlike traditional methods, a CNN model, once developed, could assess complex copper layout patterns in a cost-effective and computationally efficient manner.