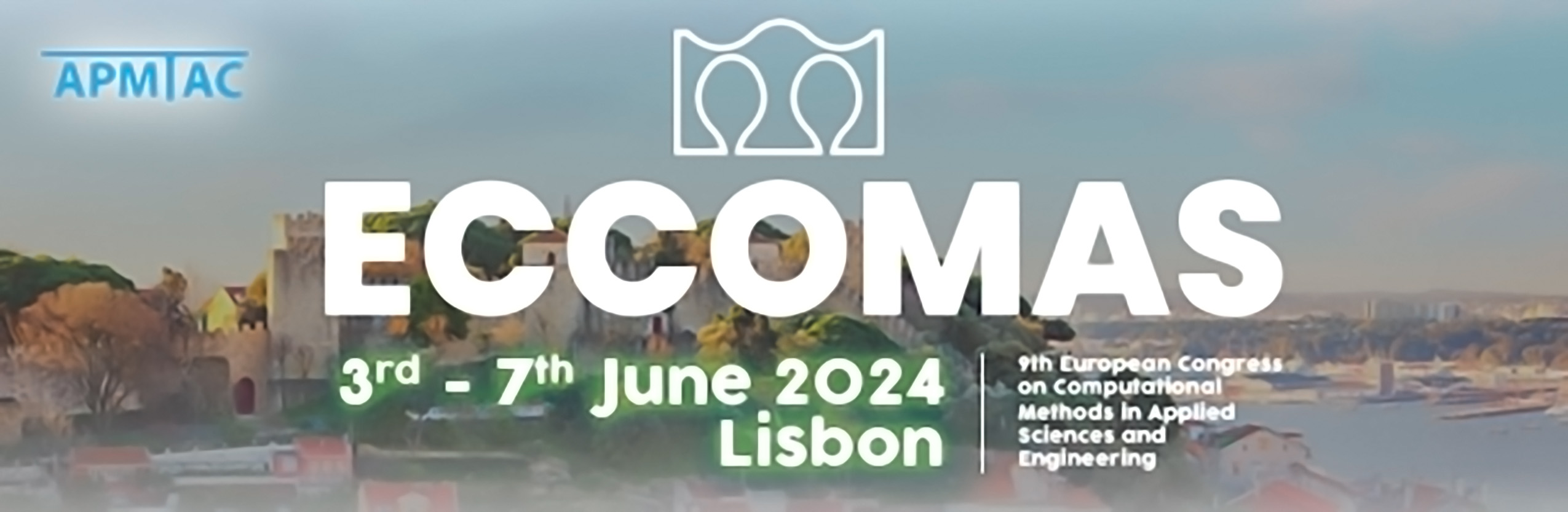
Data-assimilation closure for low-dimensional fluid models
Please login to view abstract download link
The high computational costs involved in fully resolved turbulent flow simulations form a major challenge that has prompted the development of accurate and computationally affordable simulation strategies. In large-eddy simulation (LES) a filtered description of the dynamics determines that only the largest, most energetic scales of the flow are fully resolved. Unresolved dynamics are modeled via an LES (or sub-filter) model. In this work, we develop a low-dimensional data-driven stochastic closure for fluid models by combining LES and the 3D-Var data-assimilation algorithm. We will present an efficient method for accurately predicting complex dynamics by combining a coarse numerical simulation with a data-driven term, as is commonly done in data assimilation \cite{grewal2014}. We require that the resolvable flow scales on average attain the energy value as measured from high-fidelity data, which imposes a constraint on the Fourier coefficients of the numerical solution. By adding a data-driven stochastic feedback control term \cite{blomker2013} to the evolution of these coefficients, the flow is accurately steered towards the desired energy values whilst leaving few tunable parameters. Physically, this means that the effect of small, unresolved scales on the resolved scales are modeled as stochastic processes. The approach is demonstrated for two-dimensional Rayleigh-B\'enard convection \cite{ephrati2023rb}. If time permits, we discuss the possibilities of extending this approach from the Fourier representation to general orthogonal bases.