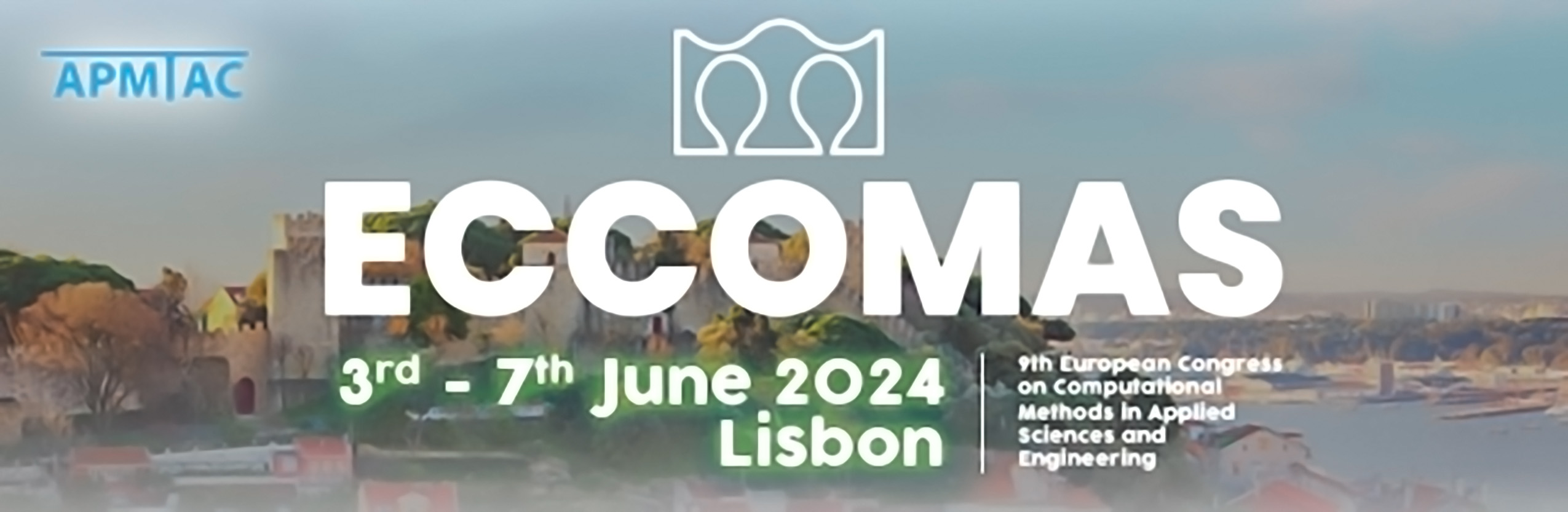
Comparison of Data-Driven Output-Only Methods for Damage Detection with a Focus on Deep Learning Algorithms
Please login to view abstract download link
Railway bridges are subject to high dynamic loads caused by the crossing of trains, which can result in structural damage to the supporting structure. In addition, external influences can affect the durability of the structure and materials [1]. In this context, the determination of the dynamic characteristics is a key element to monitor the system behavior and to derive conclusions on the service life of the structure [2]. Continuous monitoring of the dynamic behavior enables early detection of damage and thus predictive maintenance for sustainability [3]. In the context of Structural Health Monitoring (SHM), output-only methods such as Operational Modal Analysis (OMA) or the Stochastic Subspace Identification (SSI) [4, 5] are of particular interest since they can be operated continuously and cost-effectively. The Frequency Domain Decomposition method (OMA technique) enables the estimation of natural frequencies and eigenmodes of the structure based on time histories (velocities, accelerations) [6]. Damage can be detected as a result of a change in the modal parameters [2]. However, these methods sometimes do not allow accurate conclusions to be drawn about the structural damping of the structure or the severity of the damage [7]. This paper compares different output-only methods in terms of their prediction accuracy, with a special focus on deep learning algorithms [e.g. 8] (end-to-end). First, a numerical model is analyzed that allows a clear specification of the characteristics of the structure. In addition, this model is used to generate a synthetic data set that labels the degree and location of damage, as well as the damping characteristics for Structural Health Monitoring. In a further step, the presented methods are evaluated on the basis of field measurement results.