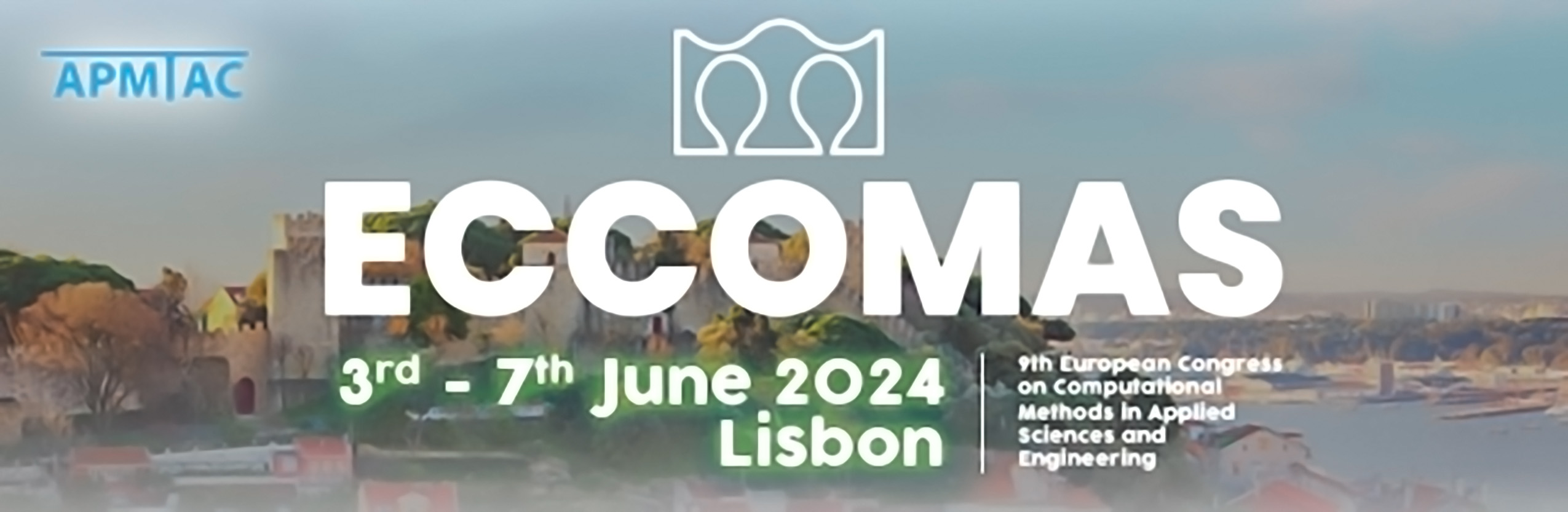
Application of Graph Neural Networks to Predict Hydrogen Explosions in Congested Environments
Please login to view abstract download link
Deflagration poses a ubiquitous risk across various industries. To ensure safety in industrial processes, its consequences need to be comprehended and mitigated, but traditional computational fluid dynamics (CFD) simulations often demand extensive computing resources and time. We illustrate an application of graph neural neural networks (GNNs) to predict the pressure, temperature and velocity fields induced by the sudden explosion of stoichiometric mixtures of hydrogen and air in complex and partially confined environments. We simulate, via URANS analyses in OpenFOAM, the ignition of a premixed flammable mixture of air and hydrogen contained in small subdomains of geometrically complex domains; the ignition induces the propagation of a deflagration front and complex transient flow conditions due to the complex and congested nature of the domain’s geometry. The turbulent combustion implies faster mixing in time, resulting in increasing flame speed and pressure wave intensity. The CFD simulations are validated by comparison with experimental data. The time history of the fields of pressure, temperature and velocity, as well as of additional variables quantifying the details of combustion and turbulence are recorded to serve as a training database. In the training simulations, we model cubic volumes of air enclosed by rigid walls, comprising multiple rigid obstacles of random shape, position and orientation, and multiple flammable domains. At time 0 one or more ignitions of the flammable mixture are performed. The ensuing deflagration is recorded in detail. A recently proposed GNN model is extended and adapted for it to learn to predict the flow field initiated by the deflagration. During the training the model learns the evolution of thermodynamic quantities in time and space, as well as the effect of the boundary conditions. After training, the model can very quickly compute such evolution for unseen geometries and arbitrary initial and boundary conditions, exhibiting good generalization capabilities for domains larger than those used in the training simulations, allowing savings in computational time of at least two orders of magnitude.