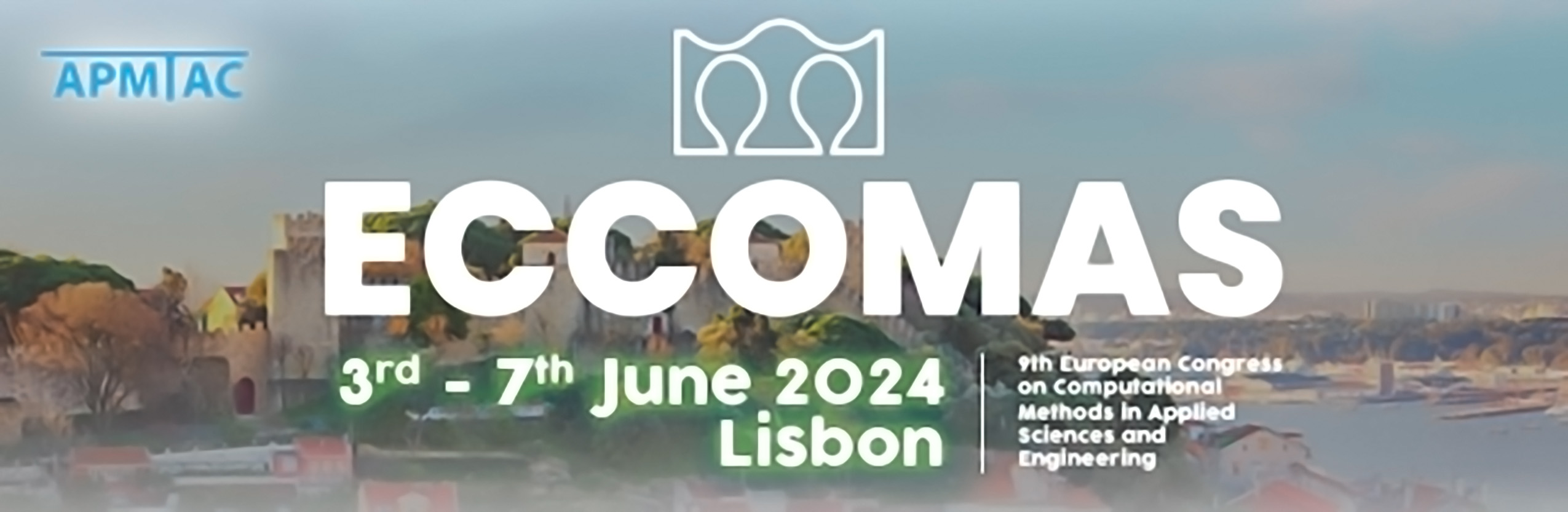
All-at-Once and Reduced Calibration Approach in Computational Solid Mechanics
Please login to view abstract download link
The calibration of constitutive models is an essential task in computational solid mechanics to ensure reliable simulation results. During model calibration, the material parameters of the constitutive model are determined based on experimental data. This is frequently carried out using the non-linear least-squares method. Other available methods are, among others, Bayesian approaches or the virtual fields method, when full-field displacement data are available. The parameter identification is often performed following the so-called reduced approach, where the state, such as displacements or reaction quantities, is expressed as a function of the sought parameters. This results in an optimization problem in the material parameter domain. In contrast, the all-at-once approach can be chosen, wherein the state and the parameters are jointly estimated, leading to an optimization problem in a much higher parameter space compared to the reduced approach. An advantage of the all-at-once approach is the elimination of particular assumptions on the model equations yielding the state. Currently, both approaches are mainly considered in the field of inverse problems, see, for example, [1]. In this contribution, the all-at-once and reduced calibration approach are applied in the context of model calibration in computational solid mechanics. First, the underlying equations of both approaches are derived considering different classes of constitutive models, such as linear small-strain elasticity, non-linear elasticity, and inelasticity. Then, both approaches are compared with first numerical results using experimental full-field data to investigate the accuracy of the calibrated material parameters and numerical expenses. To solve the underlying mathematical model, we use finite elements and physics-informed neural networks. The latter are a neural network-based framework for solving partial differential equations incorporating physical knowledge during the training process and can be used for both calibration approaches [2]. The calibration is performed in a frequentist setting with the maximum likelihood method and with a stochastic approach using Bayesian inference. [1] B. Kaltenbacher, Regularization based on all-at-once formulations for inverse problems. SIAM J. Numer. Anal., Vol. 54(4), 2016. [2] D. Anton and H. Wessels, Physics-informed neural networks for material model calibration from full-field displacement data. arXiv preprint. arXiv:2212.07723, 2022.