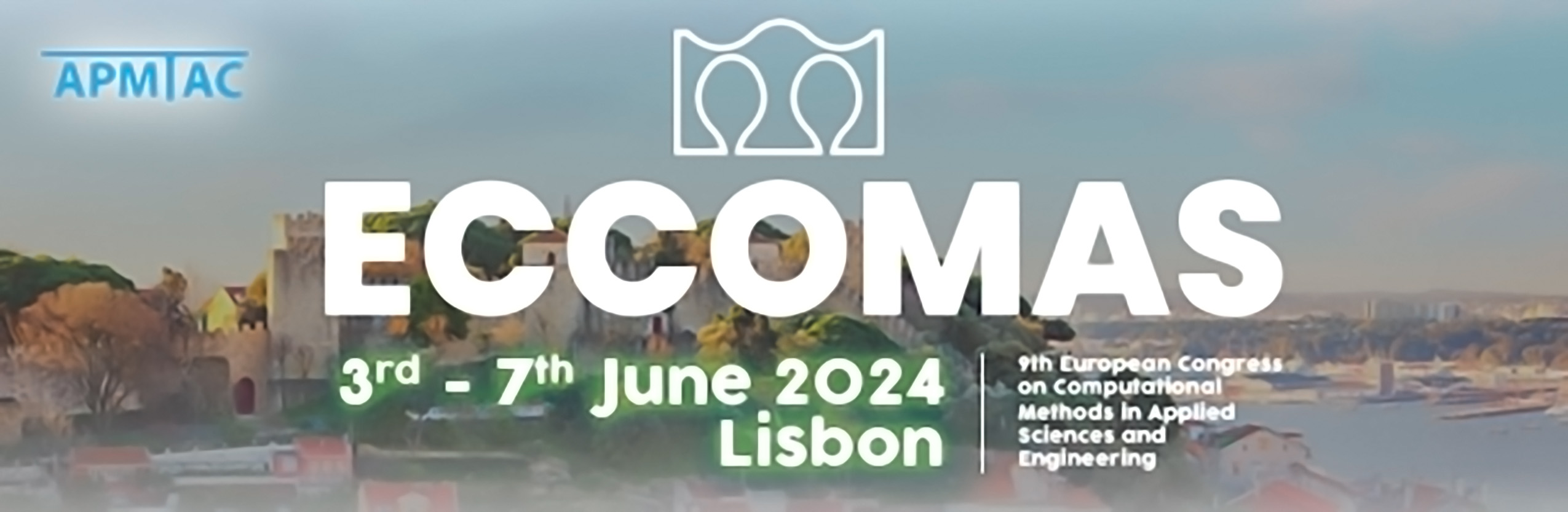
Numerical Design of Engineering Components and their Production Processes
Please login to view abstract download link
Numerical optimization is an important tool in both the design of individualized components and their manufacturing processes. Despite this vital role of numerical optimization, the creative aspects of the design process are still left to the human designer. In fact, for centuries, creativity has been considered a purely human attribute, if not the defining element of a human being. Recent advances in artificial intelligence (AI) have shaken this perception to its roots. Bit by bit, creative, rational agents have made their way into our daily lives. Inspired by these advances, we will explore different ways in which machine learning algorithms can help improve numerical design, e.g., in terms of geometry parameterization, surrogate models for forward simulations and optimization strategies. To this end, we show how variational autoencoders (VAE) can be used to learn low-dimensional, yet feature-rich, shape representations. A VAE can discover common properties among a variety of shapes without an explicit, human-made parametric representation of the original designs. A VAE is a neural network architecture that learns the underlying structure of a 3D shape in an unsupervised manner. It infers a latent, hierarchical representation of objects. This approach promises significant improvements in both performance and variety of shapes. The resulting geometric representation is then incorporated into a shape optimization framework.