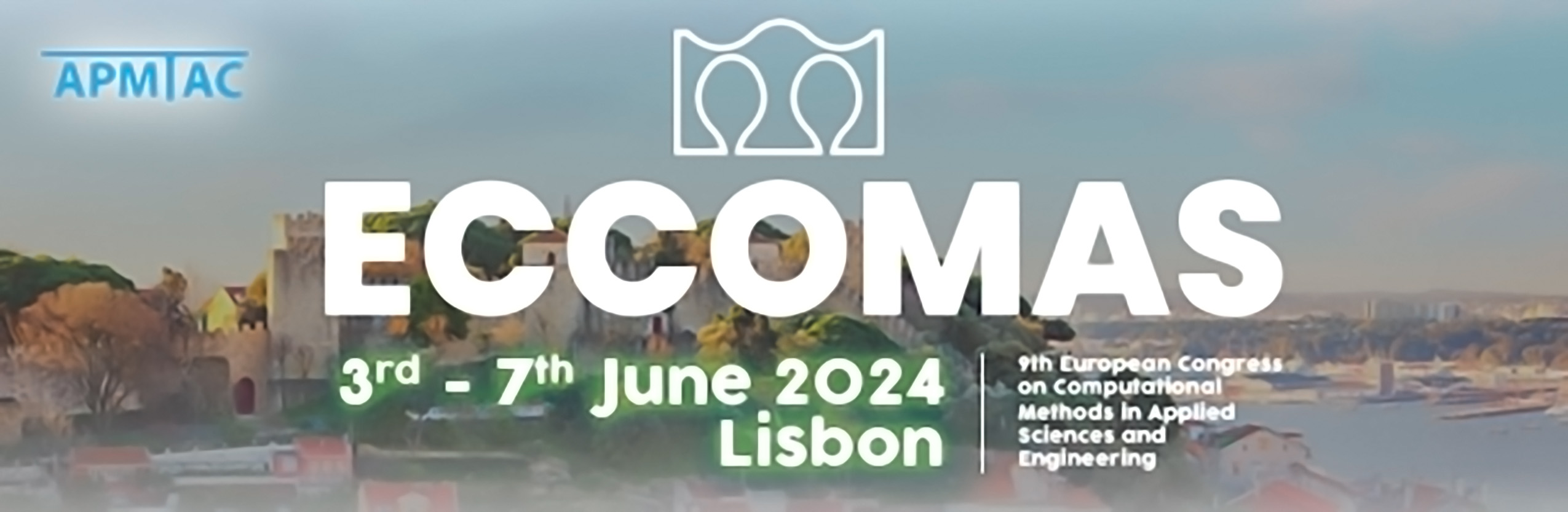
Application of an Automated Machine Learning Framework for the Preliminary Design of Engine Combustion Systems
Please login to view abstract download link
As the pursuit of decarbonization becomes progressively more challenging for internal combustion engines (ICEs), it is imperative to develop models that are not only accurate and reliable but also fast. The optimization of ICEs necessitates careful consideration of various parameters, particularly in Diesel engines where the design of the combustion chamber significantly influences both engine performance and emissions [1]. Moreover, in the automotive industry, the dual functionality of a tool as both a component design instrument and an engine calibration tool holds significant value. Traditional methods involving engine test beds are time-consuming and expensive, while 3D computational fluid dynamics (CFD) simulations are computationally demanding. In this context machine learning (ML) presents a pathway to transform the complex physical processes that occur in a combustion engine into compact informational processes [2]. This paper introduces an innovative approach that treats CFD as a virtual plant model to generate data for developing ML models. In this study, over 1000 sector-mesh CFD simulations were conducted for a heavy-duty engine. A wide range of input parameters were varied, and the CFD simulation cases were generated by sampling points from the input space. These input parameters included injector design, bowl design and various in-cylinder flow and thermodynamic conditions at intake valve closure (IVC). The outputs of interest, related to engine performance and emissions, served as targets for the simulations. Using the samples derived from CFD, ML models were trained to predict the targets based on the input features. Various ML algorithms were validated against engine CFD simulations. This research showcases the potential of ML in significantly reducing the time required for the preliminary design and calibration of engine combustion systems, all while maintaining accuracy comparable to traditional approaches.