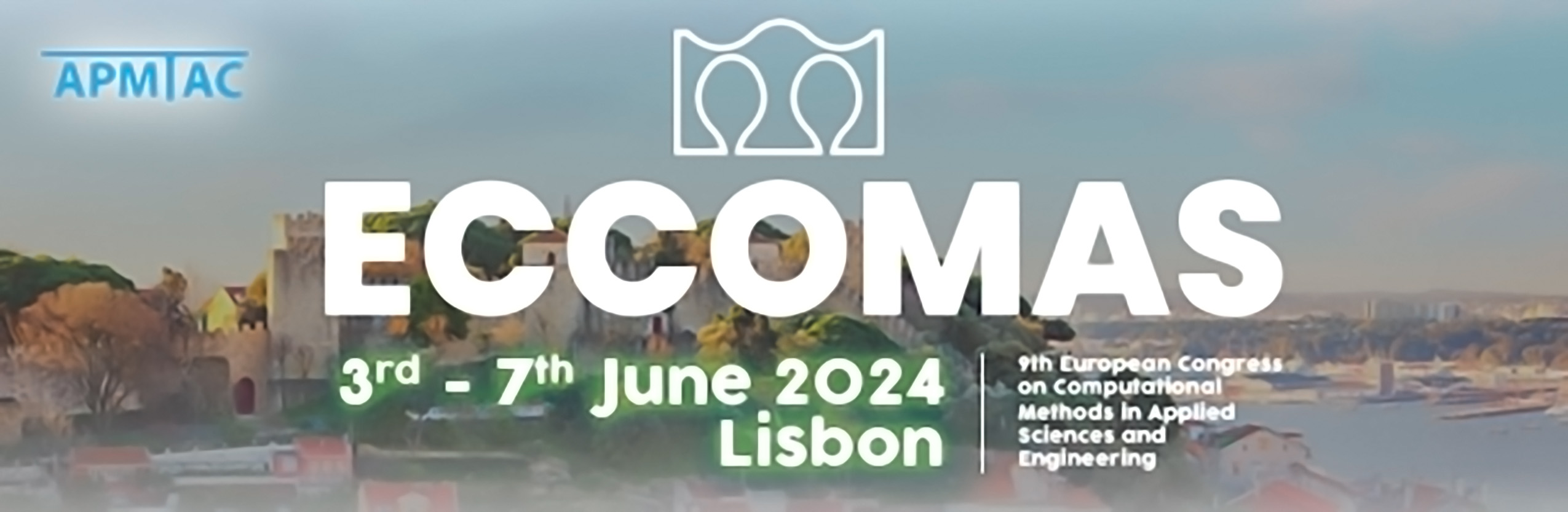
Data-Driven Extraction of Intrinsic Dynamics for Control of Continuum Robots
Please login to view abstract download link
Drawing inspiration from nature, where evolution has cultivated continuum mechanisms in animals, the next generation of robotics is poised to revolutionize our interaction with the environment. These continuum robots offer unparalleled advantages, such as increased workspace for dexterous movements, flexibility, and compliance, making them ideal robots to deploy for high-stakes applications like surgery, and deep-sea and space exploration. Unfortunately, these advantages come at significant cost, namely that these robots are high-dimensional (theoretically, infinite-dimensional), exhibit complex dynamics, and are highly nonlinear (e.g., geometry, material, friction, and contact). These properties hinder the application of modeling and control techniques that have been successful for deploying traditional rigid-body robots. In this work, we leverage recent advances in nonlinear dynamics to learn low-dimensional dynamical models of continuum robots for model-based control. We develop a data-driven framework to approximate the intrinsic dynamics of the robot on Spectral Submanifolds (SSMs). SSMs are low-dimensional, attracting manifolds that describe the dynamics of generic nonlinear system. We leverage SSMs as structural priors for system identification and exploit the latent space dynamics on the SSM's tangent space for model predictive control (MPC). By planning in the latent space, computation is drastically reduced, enabling real-time application of MPC. We present results on various continuum robot morphologies, both in finite-element simulation and on a real-world hardware platform. We benchmark against popular model reduction schemes such as project-based and Koopman-based approaches, demonstrating the efficacy and robustness of our approach in various challenging trajectory tracking tasks. Through these examples, we argue that one should seek to infer important structure between the input and output data, rather than fit to input-output data. This allows one to infer faithful models that are useful for control and can be reliably field-deployed on continuum robots operating in safety-critical and high-integrity environments.