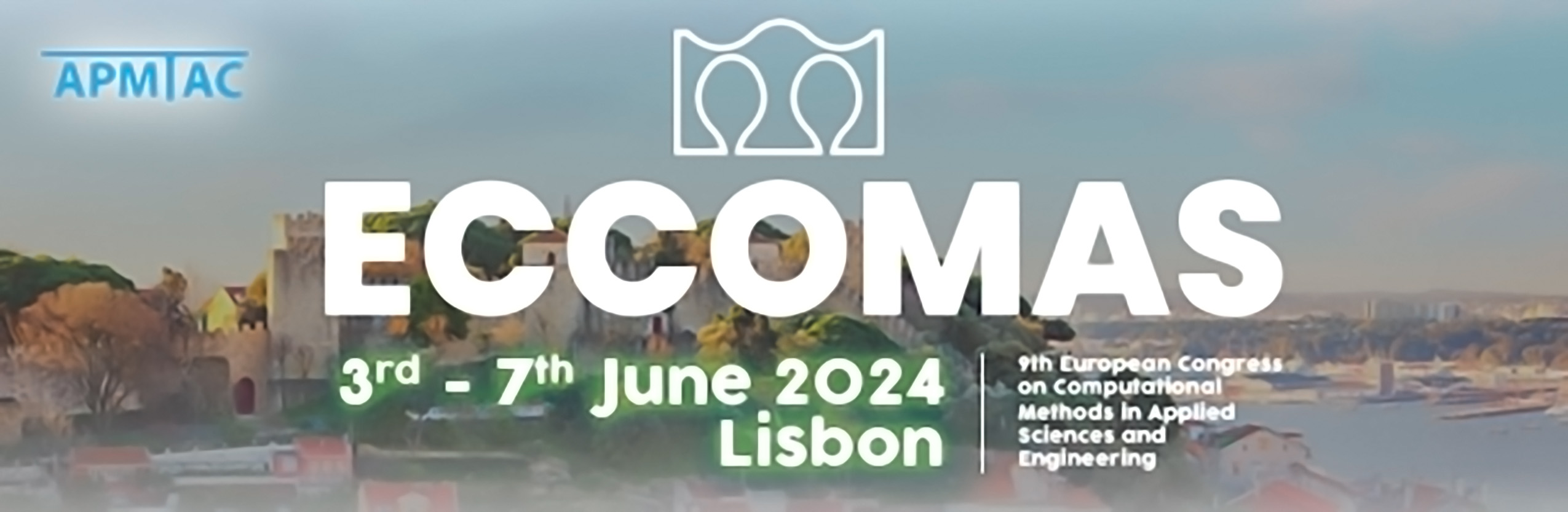
Data-Driven techniques for spatio-temporal dynamics of Turing and oscillatory type
Please login to view abstract download link
In this talk we consider Reaction-Diffusion PDE (RD-PDE) systems whose solutions present spatio-temporal dynamics of Turing and oscillatory type. We focus on the application of Dynamic Mode Decomposition (DMD) [1], an equation-free method that aims at reconstructing the best linear fit from temporal datasets. In [2], it is mentioned that DMD may fail in the case of RD–PDE systems. We show that DMD does not provide accurate approximation for datasets describing oscillatory dynamics, like spiral waves, relaxation oscillations and spatio-temporal Turing instability (see e.g. [3]). We propose a piecewise version of DMD (pDMD) to overcome this problem. The main idea is to split the original dataset in N submatrices and then apply the randomized DMD method [4] in each subset of the obtained partition. Numerical experiments show that very accurate reconstructions are obtained by pDMD for datasets arising from time snapshots of certain RD-PDE systems, like the FitzHugh-Nagumo model, a λ-ω system and the DIB morpho-chemical system for battery modeling.