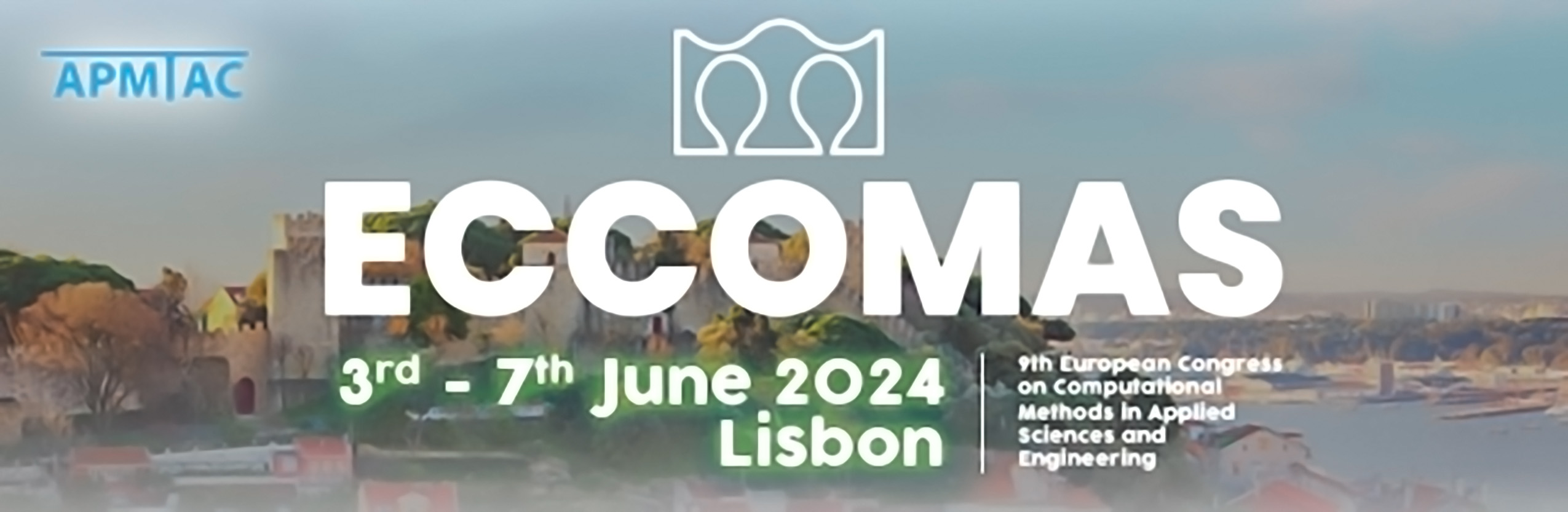
Uncertainty-aware Transonic Flow Predictions with Bayesian Deep Learning
Please login to view abstract download link
Transonic flows poses unique challenges and exhibits distinctive phenomena due to the interaction of subsonic and supersonic flow regions. One notable characteristic is the formation of shock waves, abrupt changes in pressure and density, which play a crucial role in transonic aerodynamics. High-fidelity computational fluid dynamics (CFD) simulations can provide valuable insights into such flows. Nevertheless, the prohibitive costs associated with these simulations limit their feasibility. There is a need for aerodynamic data models that possess both prediction speed and accuracy, ensuring the ability to confidently predict viable design alternatives. The objective of this work is to apply such a Bayesian deep learning model to steady-state high-fidelity CFD simulations from a 3D geometry representative of a commercial aircraft.