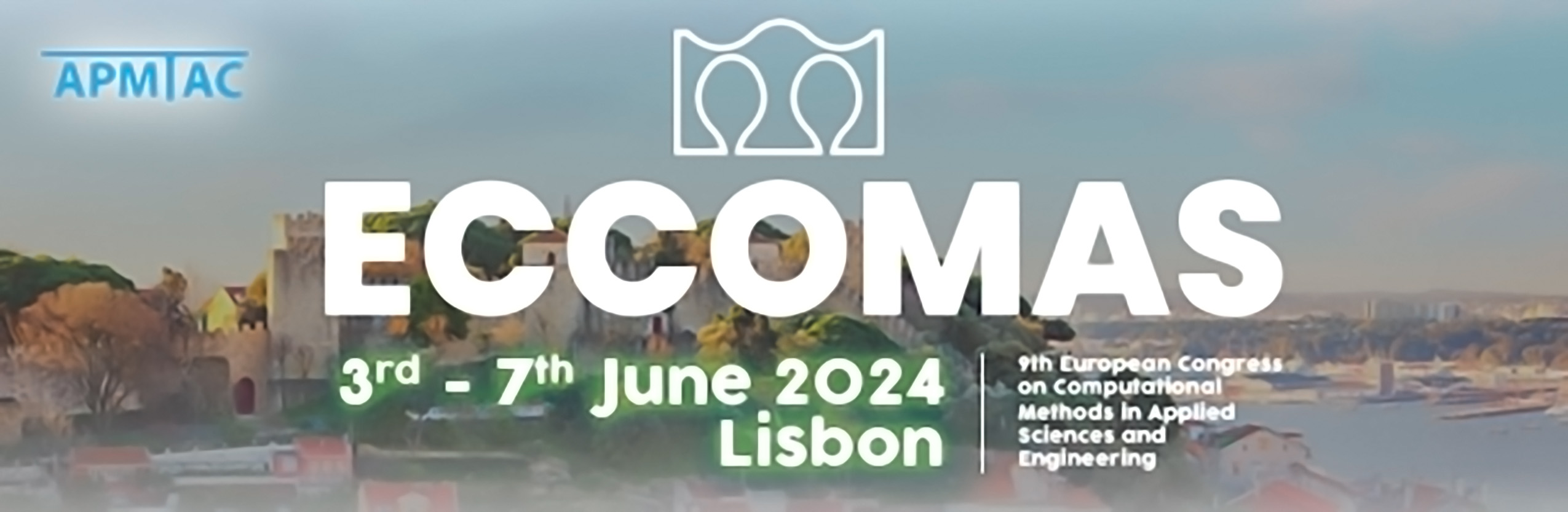
Evaluating the Probability of Infection in a UK Hospice through CFD Data Driven AI
Please login to view abstract download link
A Hospice is a place where vulnerable patients with extremely weak immune systems remain in care, therefore preventing indoor virus transmission becomes crucial. This study aims to establish a computational framework to utilise CO2 control demand ventilation (CDV) often used to open the operable windows in these spaces, to automatically mitigate cross-infection risk through natural ventilation. To achieve this, this study first proposes and employs a ‘probability of infection’ (POI) metric. This is to quantify the risk of infection and is achieved by utilising computational fluid dynamics (CFD) and the Eulerian-Lagrangian model. Additionally, the simulations employ models for the exhaled CO2. It was found that the distribution of the CO2 concentration shares similarities to that of airborne infectious particles, but there are differences. This study demonstrates that the new metric should be used to quantify and assess the risk of cross-infection instead of the widely used CO2 concentration. Using data driven from the CFD computations, an optimised random forests regression (ORF) model, using the CO2 concentration and the supply ventilation rate as inputs, was trained to predict the POI determined by rigorous simulations. The model prediction boasted a coefficient of determination of 0.99 and a root mean square of 0.0238. Thus, a computational framework is established for the development of intelligent building systems with CO2 sensors that can automatically counter airborne infection risk by adaptively varying the ventilation rate. This can be hugely beneficial for vulnerable spaces such as a Hospice