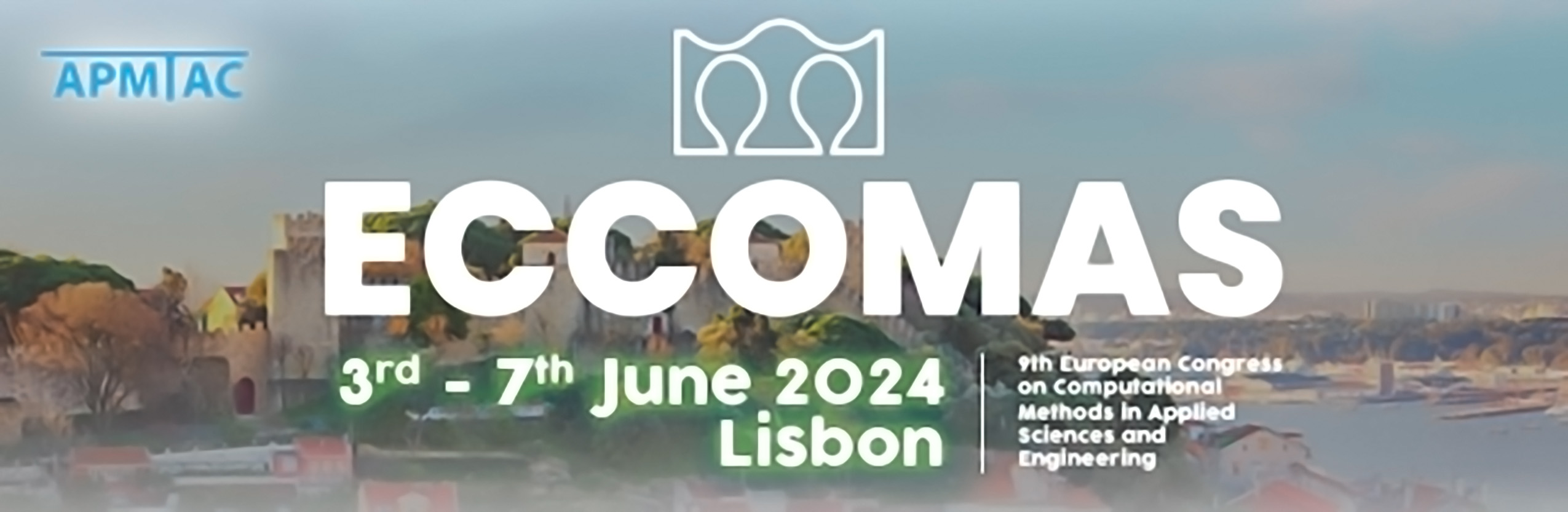
Data-driven subgrid-scale models for machine-learned implicit large-eddy simulation (ML-ILES) using the differentiable JAX-Fluids framework
Please login to view abstract download link
Differentiable solvers allow to learn physics-informed machine-learning (ML) surrogate models by backpropagating gradient information through multiple integration steps of the underlying partial differential equation. We leverage automatic differentiation capabilities of our in-house finite-volume JAX-Fluids CFD framework to devise a novel and powerful data-driven subgrid-scale (SGS) model for machine-learned implicit large-eddy simulation (ML-ILES) of compressible flows. Our SGS modeling strategy is based on an optimized physics-informed deconvolution algorithm inspired by the Adaptive Local Deconvolution Method. Contrary to standard smoothness-based reconstruction strategies like WENO or TENO, we utilize neural network-based reconstruction algorithms for each physical quantity separately such that underresolved turbulent structures are best approximated. Hereby, the deconvolution process is enriched by latent information of the encoded local flow state. The data-driven model is trained on coarse-grained spatio-temporal trajectories of direct numerical simulations (DNS). First, we apply the novel SGS model to homogenous isotropic turbulence at low turbulent Mach numbers and demonstrate that due to the chosen ML-ILES ansatz the SGS model generalizes well to various spatial resolutions, Mach numbers, and Reynolds numbers multiple orders of magnitude higher than the training data. Second, we expand the SGS model to HIT at higher turbulent Mach numbers at which eddy shocklets are present in the flow.