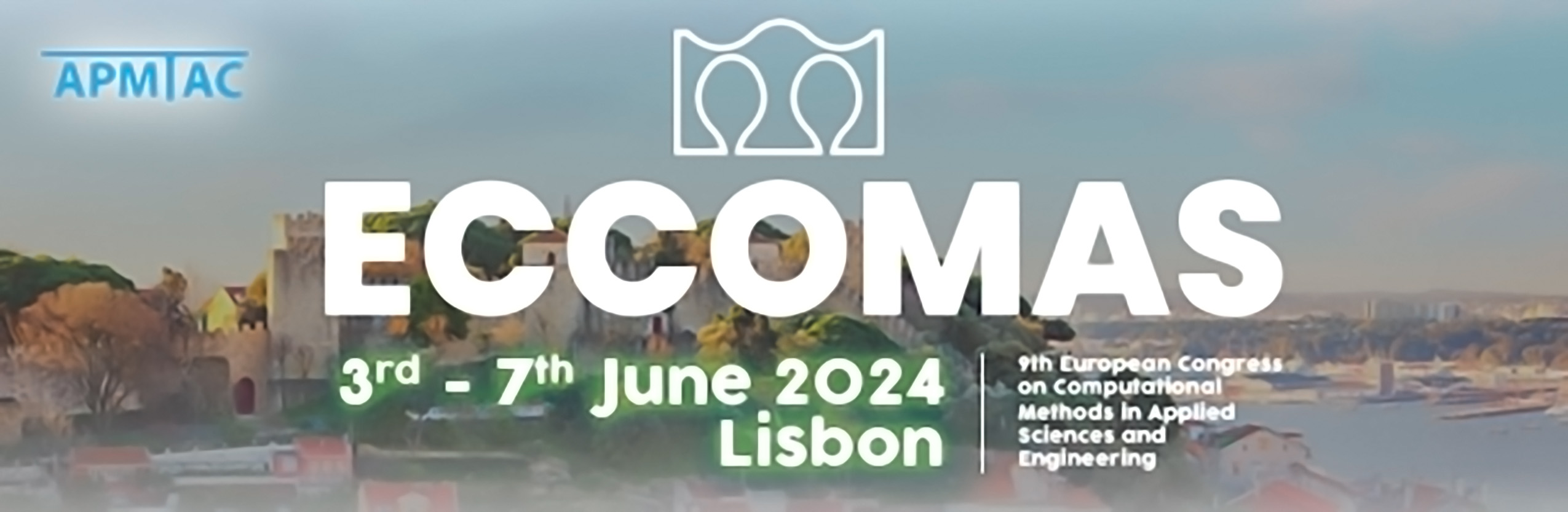
Data-Driven Nonlinear Model Reduction to Spectral Submanifolds
Please login to view abstract download link
I discuss an emerging dynamical-systems-based alternative to neural networks in the data- driven reduced-order modeling of nonlinear phenomena. Specifically, attracting spectral submanifolds (or SSMs, for short [1]) provide very low dimensional attractors in a broad family of physical problems that range from structural vibrations to transitions in fluid flows. These invariant manifolds are tangent to slow invariant subspaces of the linearized dynamical system at a hyperbolic fixed point. There are infinitely many such SSMs, but there is precisely one that is smoother than all the other ones. The dynamics restricted to this snoothest, primary SSM provide an exact reduced model for the full system, as all nearby trajectories synchronize with the SSM-reduced dynamics exponentially fast. These results enable the reduced modeling of autonomous, time-periodic and time-quasiperiodic dynamical systems purely from trajectory data [2]. Recent advances have also revealed the significance of secondary (or fractional) SSMs with lower degrees of smoothness. Indeed, it turns out that training trajectories obtained from experimental data are with probability one on fractional SSMs, necessitating the use of fractional-powered expansions in reduced-order modeling. Further recent progress in SSM theory includes its extension to non-autonomous dynamical systems with arbitrary, temporally aperiodic time dependence. I illustrate SSM-based reduced modeling on several numerical and experimental data sets from structural vibrations, fluid sloshing, hydrogel oscillations, transitions in plane Couette and pipe flows, as well as in the model-predictive control of soft robots. REFERENCES [1] G. Haller and S. Ponsioen, Nonlinear normal modes and spectral submanifolds: existence, uniqueness and use in model reduction. Nonlinear Dynamics 86: 1493-1534, 2016. [2] M. Cenedese, J. Axås, B. Bäuerlein, K. Avila & G. Haller, Data-driven modeling and prediction of non-linearizable dynamics via spectral submanifolds. Nature Communications. 13:872, 2022, [3] Nonlinear model reduction to fractional and mixed-mode spectral submanifolds. Chaos 33 [4] G. Haller and R. Kaundinya, Nonlinear model reduction to temporally aperiodic spectral submanifolds. Chaos, submitted, 2023.