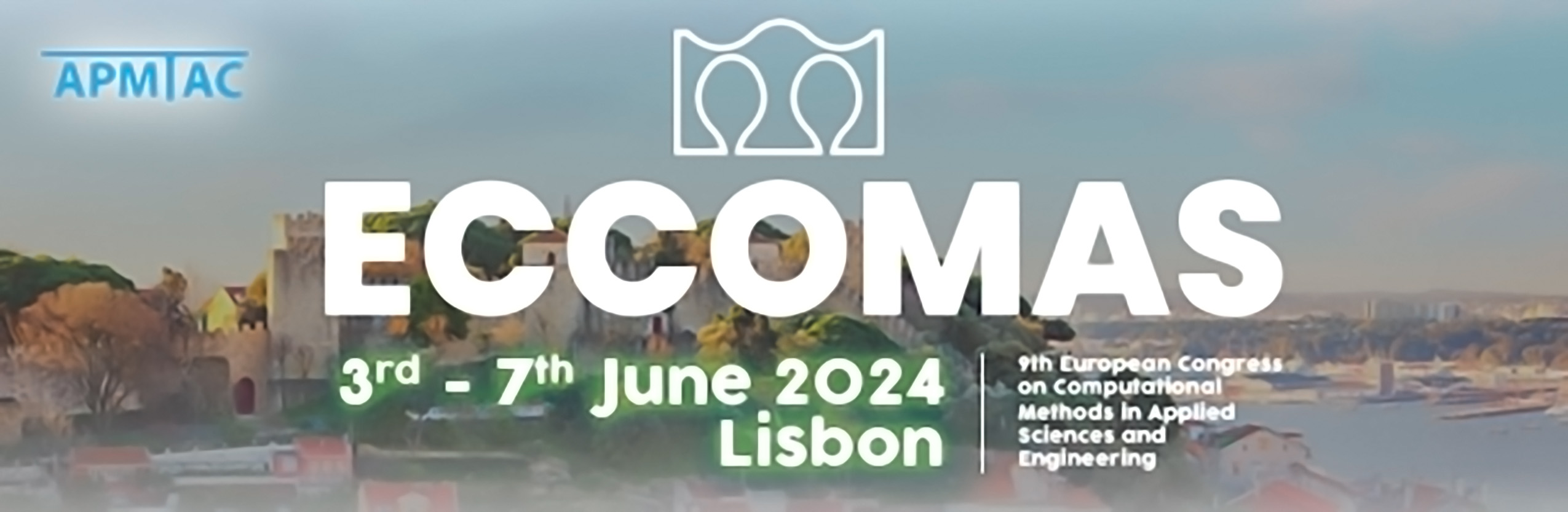
Machine Learning-Accelerated Predictions of Design Allowable of Composite Laminates
Please login to view abstract download link
The generation of design allowables for composite laminates is of utmost importance for the design and certification of the composite structures used in the aerospace industry. The determination of these design allowables, usually relies on expensive and time-consuming experimental test campaigns. With the increase of computational power, and the development of high-fidelity numerical models that accurately represent the response of composite materials, alternatives to generate design allowables based on finite element simulations have also been sought out to reduce the certification costs. However, these solutions are still computationally expensive. The recent advances on machine learning techniques opens a new window of possibilities for the faster prediction of the structural response of materials, by allowing the definition of surrogate models that continuously and analytically describe the design space [1]. In this work, a database of open-hole high-fidelity simulations [2,3] is generated and used to train machine learning algorithms, resulting in surrogate models that are able to predict the notched strength of several materials, layups and notched geometries. The trained machine learning algorithms are shown to be able to predict the strength of open-hole multidirectional composite coupons with the precision provided by high-fidelity simulation in milliseconds, corresponding to a time-to-prediction speed-up of over 10k times.