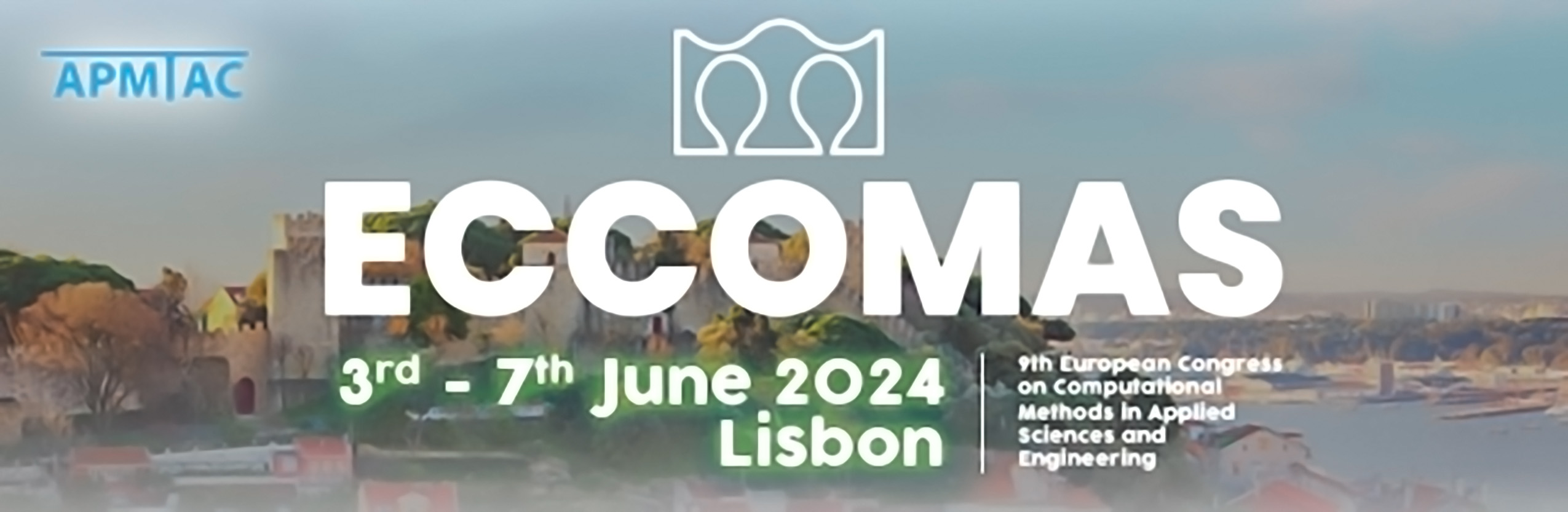
Variationally Mimetic Operator Networks
Please login to view abstract download link
Operator networks have emerged as promising machine learning tools for approximating the solution to partial differential equations (PDEs) [1,2]. Such networks map input functions that describe material properties, forcing functions and boundary data to the solution of a PDE. This work describes a new architecture for operator networks that approximates a discrete variational or weak formulation of the problem [3], such as the finite-element or isogeometric formulation, that maps discrete input functions to the discrete solution of a PDE. We apply such a variationally mimetic operator network to a canonical elliptic PDE and analyze the error in approximating the solution to the PDE. We ask the question whether a variationally mimetic architecture would lead to significant computational benefits and conclude the discussion with numerical examples. [1] Lu Lu, Pengzhan Jin, Guofei Pang, Zhongqiang Zhang, and George Em Karniadakis. Learning nonlinear operators via deeponet based on the universal approximation theorem of operators. Nature Machine Intelligence, 3(3):218–229, 2021. [2] Nikola Kovachki, Zongyi Li, Burigede Liu, Kamyar Azizzadenesheli, Kaushik Bhattacharya, Andrew Stuart, and Anima Anandkumar. Neural operator: Learning maps between function spaces. arXiv preprint, arXiv:2108.08481, 2021. [3] Patel Dhruv, Deep Ray, Michael Abdelmalik, Thomas Hughes, and Assad Oberai. Variationally mimetic operator networks. Computer Methods in Applied Mechanics and Engineering 419 (2024): 116536.