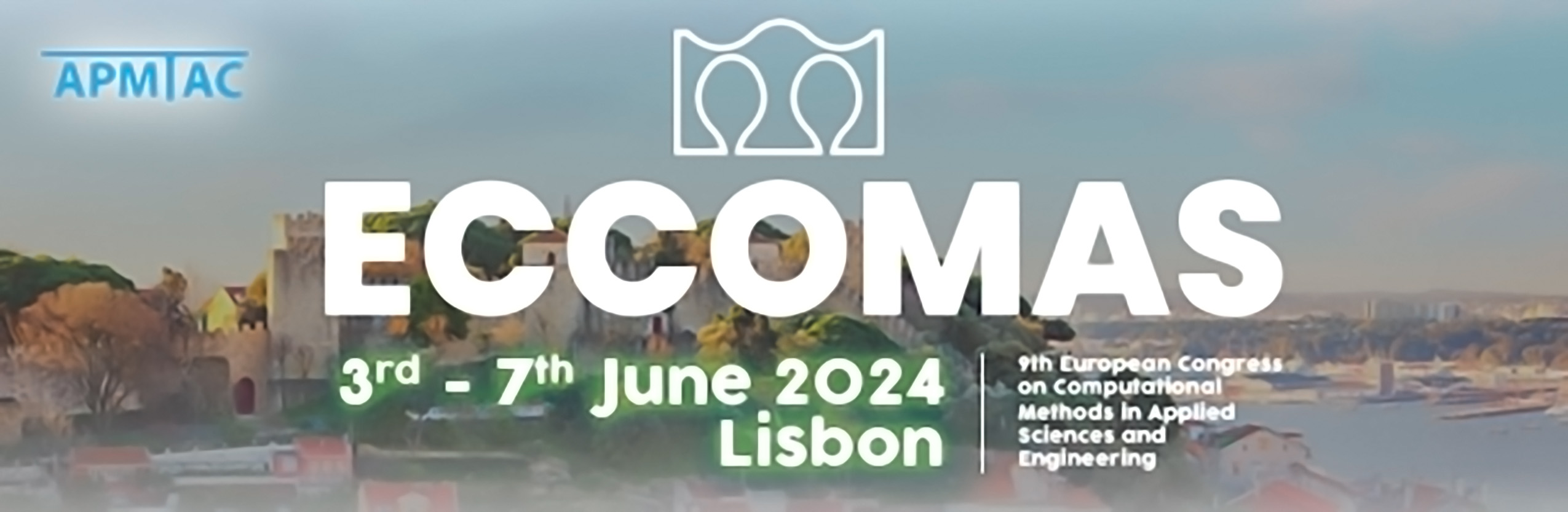
Non-intrusive reduced models for arbitrarily shaped parametric domains
Please login to view abstract download link
Real-time simulations are models that operate at speeds that nearly match real-world occurrences. These simulations rapidly process input data to provide instantaneous feedback, allowing for interactive and responsive experiences. However, training of such real-time models require the generation of numerous system responses (snapshots) which are often generated by computationally expensive high-fidelity simulations, such as finite elements or finite volume methods. The amount of training data increases significantly with the number of model inputs that are being explored. In the framework of non-intrusive reduced order modelling, the high-fidelity solvers are treated as a black-box, an attractive feature which avoids meddling with the nuances of these solvers. Methods such as Sparse-subspace learning [1] sparsely sample the parametric space for snapshot generation during the offline (training) phase while utilising hierarchical polynomial interpolation for resolving an online query. However, such methods are limited to Cartesian parametric domains, where the range of any single input parameter is independent of other parameters. In our work, the SSL framework is extended to arbitrarily shaped parametric domains. The non-Cartesian parametric domain is sampled using so-called Leja sequences, while exploring different regression techniques for high-order polynomial regression such as spline energy minimization and Ridge regression, etc. A novel sampling strategy includes a modified Leja sampling with a residual-weighed distance function. Nested polynomial interpolants ensure that interpolation can be enriched with new samples at any stage without recomputation from scratch. The work is funded by the French region of Pays de la Loire.