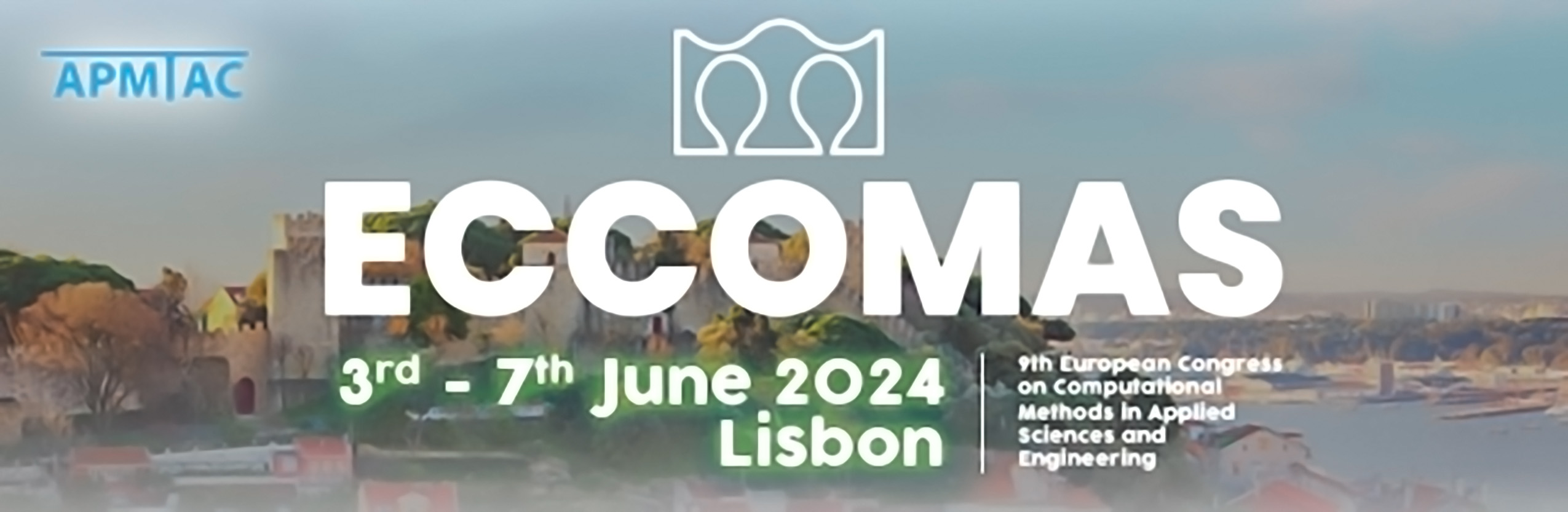
A physical-informed FE-NN methodology for predicting highly nonlinear thermomechanical response of thermoset and thermoplastic polymers
Please login to view abstract download link
Polymers are recognized for enabling the design of lightweight, advanced composites that combine cost-effectiveness with high performance properties. Nowadays, they are used in various engineering fields including aerospace, automotive, energy or medicine, and others. There is a wide variety of polymers, each exhibiting complex non-linear thermomechanical behaviour that must be investigated and characterized to determine their range of applicability. To characterize the dependencies on temperature, strain-rate, and pressure, a wide range of constitutive models have been developed to identify their diverse nonlinear behaviour. However, all these models are only operative for a specific set of parameters merely valid in narrow ranges or under particular loading conditions, leading to discontinuity and inconsistency in the utilization of the model. Moreover, experimental constraints under extreme loading rates and temperatures pose challenges in calibrating model parameters. Presently, the capabilities of AI-based techniques bring opportunities to support the development of constitutive models as well as the understanding, identification and characterization of their parameters leading to meaningful and physical-based mechanical responses consistent with the existing data. In this work, we propose a novel, unified Neural Network (NN)-based material model that captures the rate-, temperature- and pressure-dependent behaviour of polymers under monotonic loading conditions. The power of the proposed unified NN relies on requiring just a minimal training dataset obtained from straightforward simulations, encompassing both tensile and compression tests across three loading rates and three temperatures. After the dataset preparation, a Fully-connected Neural Network (FNN) is constructed to learn the constitutive relationships that rely on physical-based concepts. The trained NN-based model is implemented in the Finite Element Method (FEM), creating a hybrid FE-NN scheme for simulating the thermomechanical response of arbitrarily complex structures under different loading and environmental conditions not considered during the training process. The figure below presents a simplified overview of this approach.