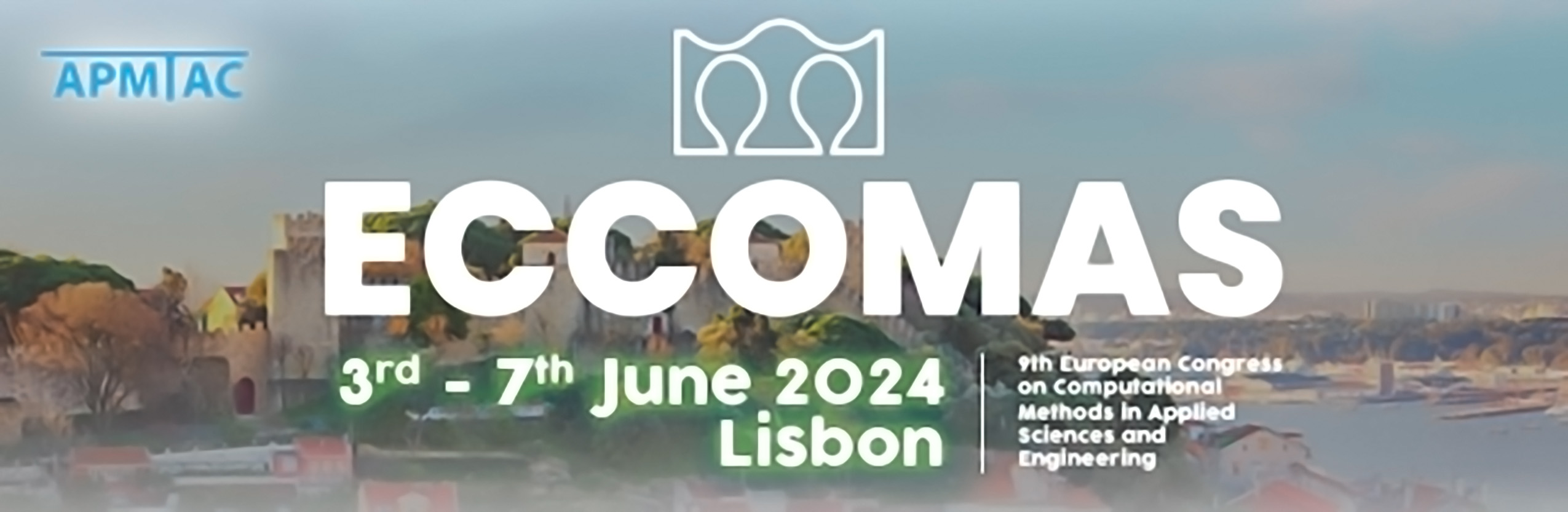
Parameter estimation in cardiac models using physics-informed neural networks
Please login to view abstract download link
The research community is making rapid strides in advancing the development of cardiac biophysical models for clinical applications, owing to their predictive capabilities and their role in facilitating the interpretation of clinical data. Nevertheless, the computational expense associated with high-resolution and accurate multi-physics models, coupled with the intricate calibration of numerous parameters (some of which may be heterogeneous in space), poses challenges for their translation into clinical settings. This work introduces an novel methodology integrating physics-informed neural networks (PINNs)\cite{Raissi} with three-dimensional nonlinear biomechanical models of soft tissue, with a particular focus on cardiac models. This approach enables the reconstruction of displacement fields and the estimation of heterogeneous patient-specific biophysical properties. The proposed learning algorithm utilises information from a limited dataset of displacement and, in certain cases, strain data routinely acquired in clinical settings. It combines this data with the physics of the problem, represented by a mathematical model based on partial differential equations, to regularise it and thus enhance convergence properties. Several benchmarks are presented to highlight the accuracy and robustness of the proposed method. The results underline its significant potential to enable the robust and effective identification of patient-specific, heterogeneous physical properties, such as tissue stiffness and active contractility. Notably, the study demonstrates the capability of PINNs to detect the presence, location, and severity of scar tissue, showcasing its utility in developing personalised simulation models for disease diagnosis, particularly in cardiac applications.