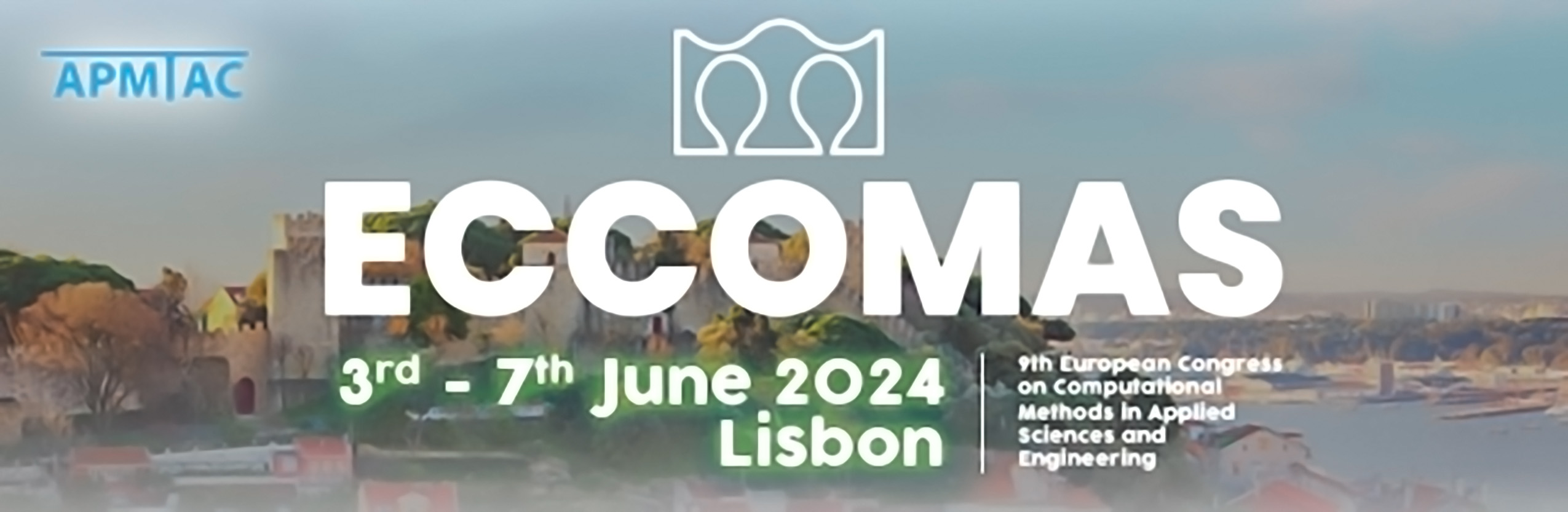
Adaptive Viscosity Methods for Turbulent Compressible Flow Computations
Please login to view abstract download link
We present a novel approach for the computation of turbulent flows. The proposed method is completely based on the numerics, but it is designed to reproduce the right physics. An automatic dissipation adjustment (ADA) method [1,2,3] is combined with the a posteriori paradigm [4], in order to obtain an implicit subgrid scale model and preserve the stability of the numerical method. The numerical scheme is designed to increase the dissipation in the under-resolved regions of the flow, and to decrease the dissipation in regions with excessive numerical viscosity. In order to keep the stability of the numerical scheme, the a posteriori approach [4] is used. It allows to increase the dissipation quickly in cells near shocks if required, ensuring the stability of the scheme. Some numerical tests are performed using different methods to highlight the accuracy and robustness of the proposed approach. REFERENCES [1] Li C-G, Tsubokura M. (2017). An implicit turbulence model for low-mach roe scheme using truncated Navier–Stokes equations. Journal of Computational Physics, 345:462–74. [2] Nogueira, X., Ramírez, L., Fernández-Fidalgo, J., Deligant, M., Khelladi, S., Chassaing, J.-C., Navarrina, F., (2020), An a posteriori-implicit turbulent model with automatic dissipation adjustment for Large Eddy Simulation of compressible flows, Computers & Fluids, 197, 104371. [3] Fernández-Fidalgo, J., Ramírez, L., Tsoutsanis, P., Colominas, I., Nogueira, X. (2021). A reduced-dissipation WENO scheme with automatic dissipation adjustment, Journal of Computational Physics, 425, 109749. [4] Clain, S., Diot, S., Loubère, R. (2011), A high-order finite volume method for systems of conservation laws- multidimensional optimal order detection (MOOD). Journal of Computational Physics, 230:4028–50