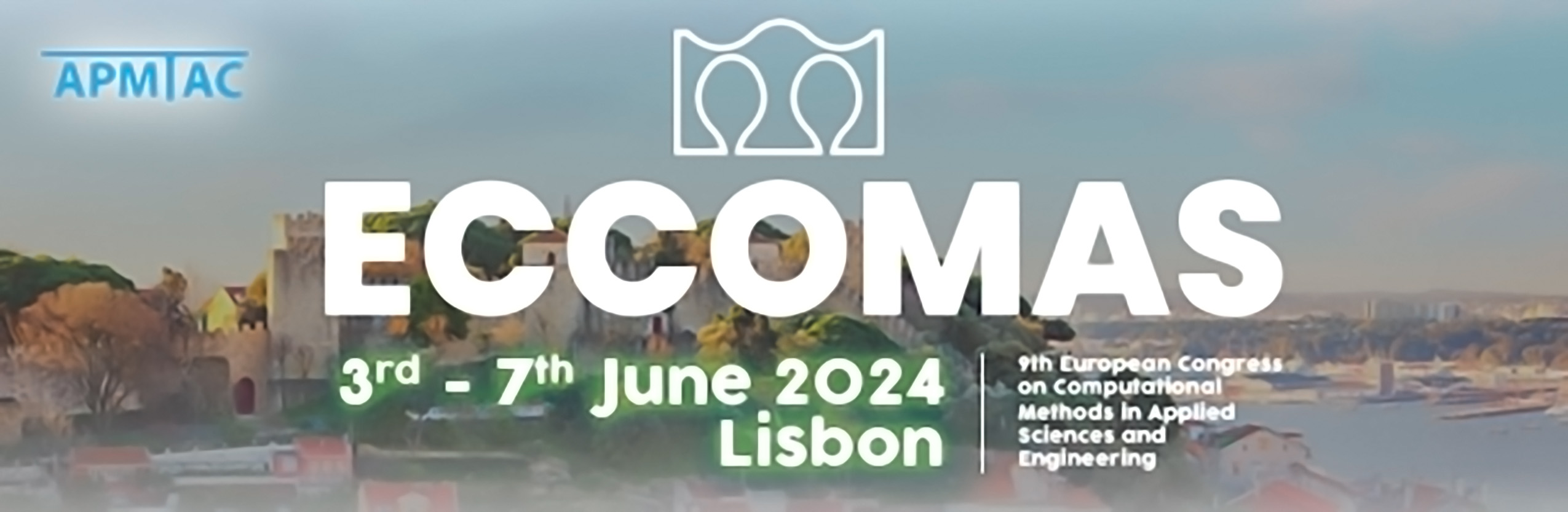
DeepONet for Matrix-Free Preconditioners Construction
Please login to view abstract download link
Preconditioning of linear systems is an essential element for guaranteeing a fast convergence of Krylov subspace methods and a very mature field of research [1, 2]. However, severe difficulties may still arise when dealing with matrix-free environments, where the system matrix is too big or too dense for being explicitly computed and stored. In this study, we propose a novel approach to approximate the application of a matrix inverse by utilizing a supervised deep learning framework called DeepONet [3] that can learn nonlinear operators through a training process on input-output vector pairs. Specifically, we implement DeepONets to approximate the action of the inverse of the Schur Complement arising in saddle-point problems, the use of which can be very effective to precondition indefinite block systems in many applications [4]. We test the proposed approach on some preliminary case problems to investigate its potential as a valid and innovative alternative to compute preconditioners for matrix-free iterative methods. REFERENCES [1] Chow, E., & Saad, Y. (1998). Approximate inverse preconditioners via sparse-sparse iterations. SIAM Journal on Scientific Computing, 19(3), 995-1023. [2] Benzi, M., & Tuma, M. (1998). A sparse approximate inverse preconditioner for nonsymmetric linear systems. SIAM Journal on Scientific Computing, 19(3), 968- 994. [3] Lu, L., Jin, P., Pang, G., Zhang, Z., & Karniadakis, G. E. (2021). Learning nonlinear operators via DeepONet based on the universal approximation theorem of operators. Nature machine intelligence, 3(3), 218-229. [4] Murphy, M. F., Golub, G. H., & Wathen, A. J. (2000). A note on preconditioning for indefinite linear systems. SIAM Journal on Scientific Computing, 21(6), 1969-1972