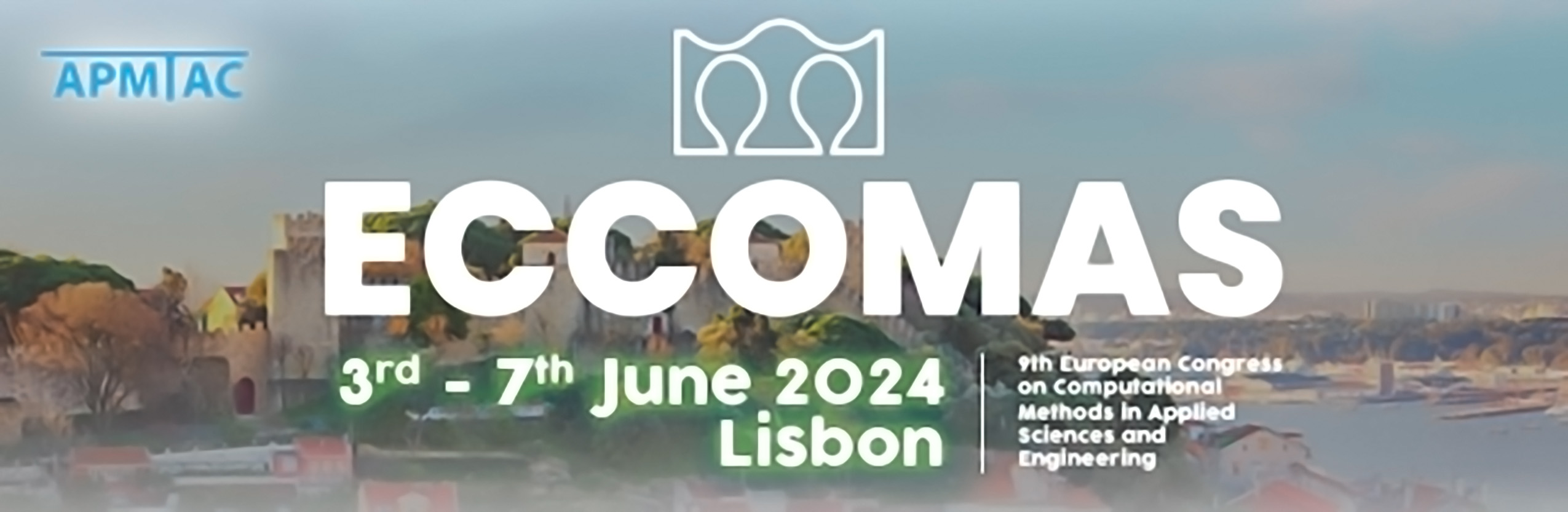
New treatment of the boundary conditions in Deep-Galerkin/PINNs methods: application to high dimensional non-linear parabolic PDEs
Please login to view abstract download link
The goal of this work is to develop robust and stable deep learning numerical methods for solving high-dimensional nonlinear parabolic PDE models by means of Deep-Galerkin/PINNs techniques. The motivation arises from the difficulty of finding and numerically imposing the boundary conditions, which are always delicate and critical tasks both in the classical numerical methods and thus also in the ANN setting. The main contribution of this work is the novel treatment of the boundary conditions in PINNs framework. The common approach consists in assigning weights to the different terms involved in the loss function, where the selection of this weights {can be done heuristically or by means of optimization procedures, see, for example, \cite{gPINNs} and the references therein}. We introduce a new idea that consists in taking into account the loss terms due to the boundary conditions by means of evaluating the PDE operator restricted to the boundaries. Although this is non feasible in the classical PDE solving algorithms, it is very intuitive within the PINNs framework since, by means of AD, we can evaluate this operator in the boundary even in the case it contains normal derivatives to such a boundary. In our approach the boundary operators are reformulated, getting rid of the choice of the boundary loss weights. Another advantage is that in this way the value of such addends is of the same magnitude of the interior losses. Although the proposed methodology is general we show the solution of PDE models for challenging problems appearing the the computational finance field. In particular, we consider the derivative valuation problem in the presence of counterparty credit risk, which includes in its formulation the so-called x-value adjustments (XVA), see \cite{burgard2011partial}.