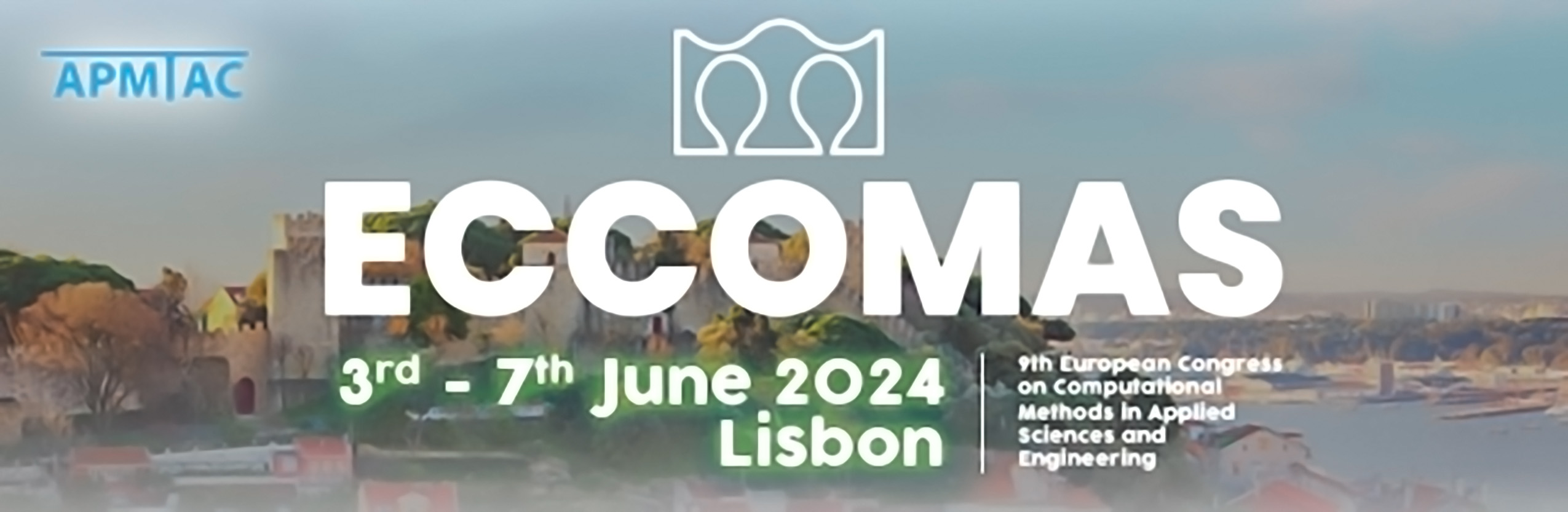
Parsimonious PINN-PGD with full domain decomposition capabilities
Please login to view abstract download link
Physics informed neural networks (PINNs) are currently investigated by the computational mechanics community to assess their potential applications in industrial context. Recent analysis shows that the use of parsimonious neural networks can improve the physical interpretability of the neural networks, as well as the final solution of the problem [1]. Furthermore, the Proper Generalized Decomposition (PGD) is recognized as an incremental construction approach with full domain decomposition potential, thereby alleviating the curse of dimensionality [2]. For example, a 3D domain can be decomposed into a sequence of 1D domains or 2D times 1D domains. In this work, we harness the potential of the proper generalized decomposition technique to develop a physics informed parsimonious neural network with domain decomposition ability. This network solves a multidimensional problem in a fully separated manner, offering parsimonious incremental possibilities while preserving domain decomposition capabilities. The loss function is computed using the separated decomposition, eliminating the need to reconstruct the full multidimensional domain. The resulting network is constructed with minimal memory usage and can be gradually improved to achieve the required precision over the residual error or the loss function.