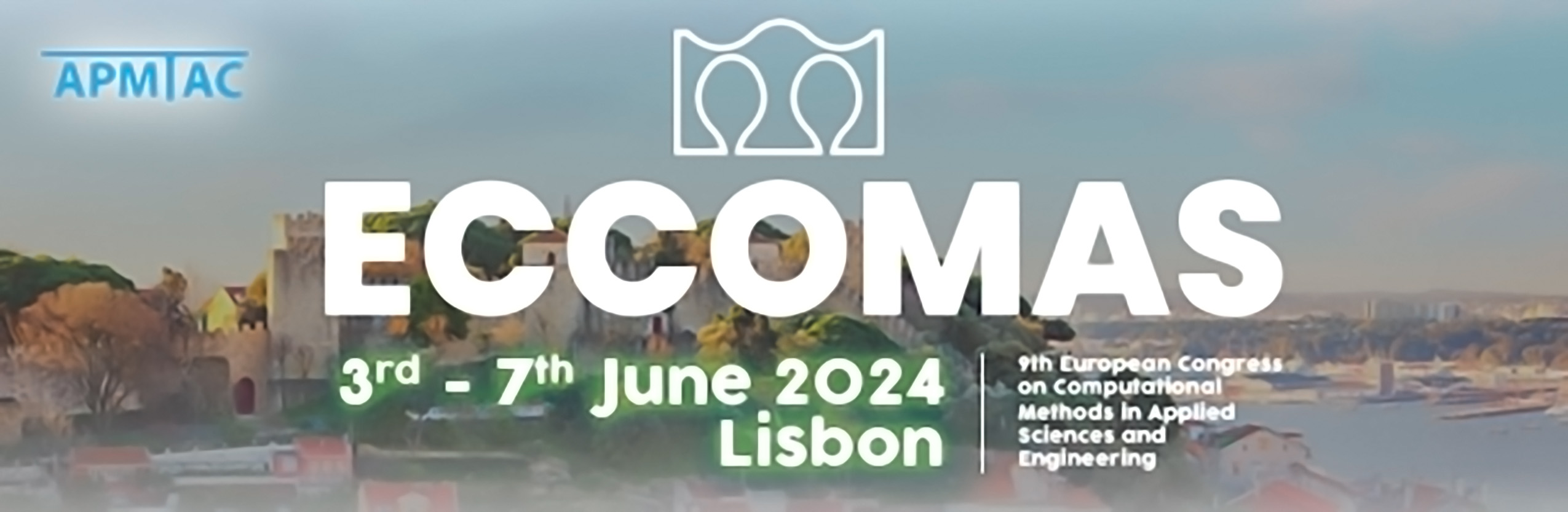
Inverse airfoil design considering uncertainties of GAN models
Please login to view abstract download link
Deep neural networks (DNNs) are recently utilized to generate desired shapes that meets requirements \cite{Yone,Yone2}. However, it is known that DNNs have uncertainties, and hence the generated shapes also contains uncertainties. In the present study, we utilize the concept of Bayesian neural network (BNN) with generative adversarial networks (GAN) to quantificate the uncertainties of generated shapes. In the proposed method, Wasserstein GAN with gradient penalty (WGAN-gp) with continuous conditional label is trained. The lift coefficient at a certain angle of attack is specified as the continuous label. The WGAN-gp network is trained using drop out technique, and then the generator network is processed with using the same dropout to output variance of the output shapes. It is noted that the computed variance is the variance of the shape, not the variance of the label. We compare the variance of the shapes and corresponding variance of the lift coefficient, and show that those two values are not the same. We also propose the method to decrease the variance by adding training dataset based on the variance distributions.