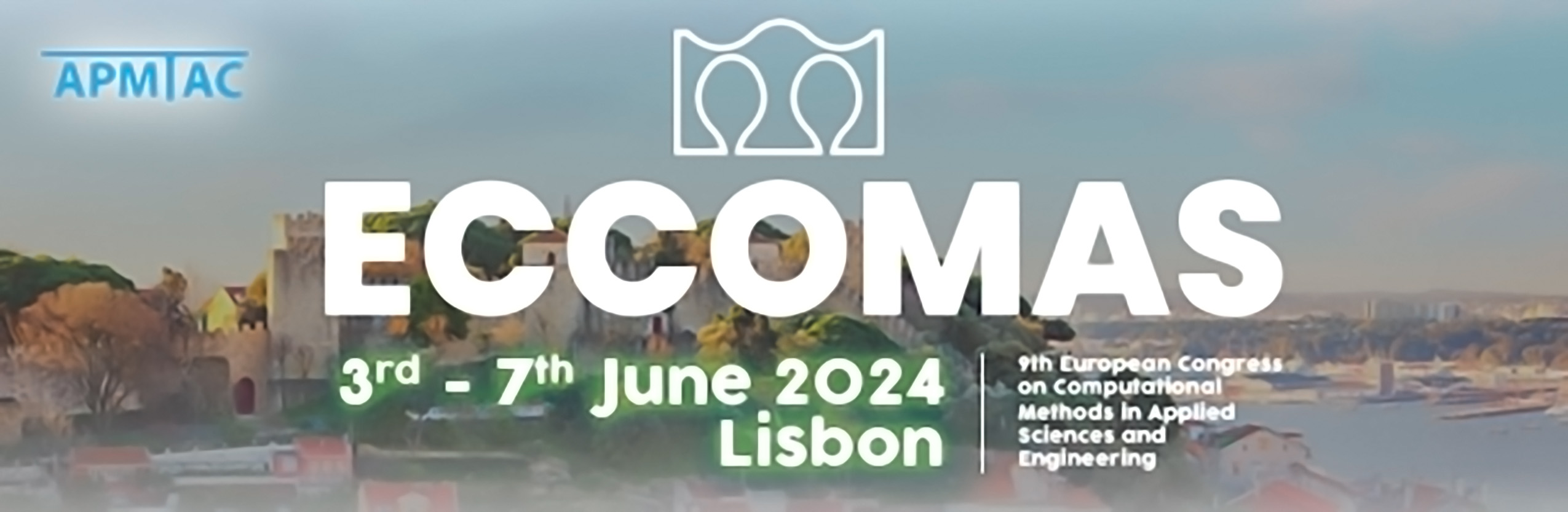
Accelerating risk averse topology optimization via importance sampling
Please login to view abstract download link
The presentation focuses on accelerating the evaluation of objectives and constraints for topological optimization of mechanical systems under uncertainties. The considered topology optimization problems are based on the so-called density approach, where the design is represented by a density field that takes the value one in the solid parts of the domains and zero in the void. The optimization goal is to distribute a specified amount of material in a design domain by minimizing a predefined objective measuring the performance of a linear elastic structure. A solution to the optimization is guaranteed by applying regularization to the problem, which smooths the density and produces intermediate density values requiring post-processing. A crisp solution is achieved with the help of a Heaviside projection step combined with erosion and dilation of the design. The latter leads to the so-called robust formulation, which minimizes the worst possible scenario. Finding the worst-performing case for simple erosion/dilation operations and objectives based on compliance is relatively easy. However, locating the worst case is far from trivial and often unnecessary for more complicated problems involving support failure, localized damage, or uncertain loads. Furthermore, designing for the worst case will enforce excessively stringent design requirements, limiting the solution space and increasing the production and operational cost of the designs. Therefore, the Conditional Value-at-Risk (CVaR) is employed to provide larger design freedom. In practical applications, CVaR is often estimated by sampling, which can lead to an extremely high computational cost as every sample in topology optimization requires the solution of a forward model representing the physics and its adjoint. To resolve the above issue, we propose utilizing the dual representation of CVaR, defined via an optimization problem over a set of probability densities called a risk envelope. In addition, we combine the dual representation with importance sampling and demonstrate the approach for 2D and large-scale 3D risk-averse topology optimization problems. This work was performed under the auspices of the U.S. Department of Energy by Lawrence Livermore National Laboratory under Contract DE-AC52-07NA27344.