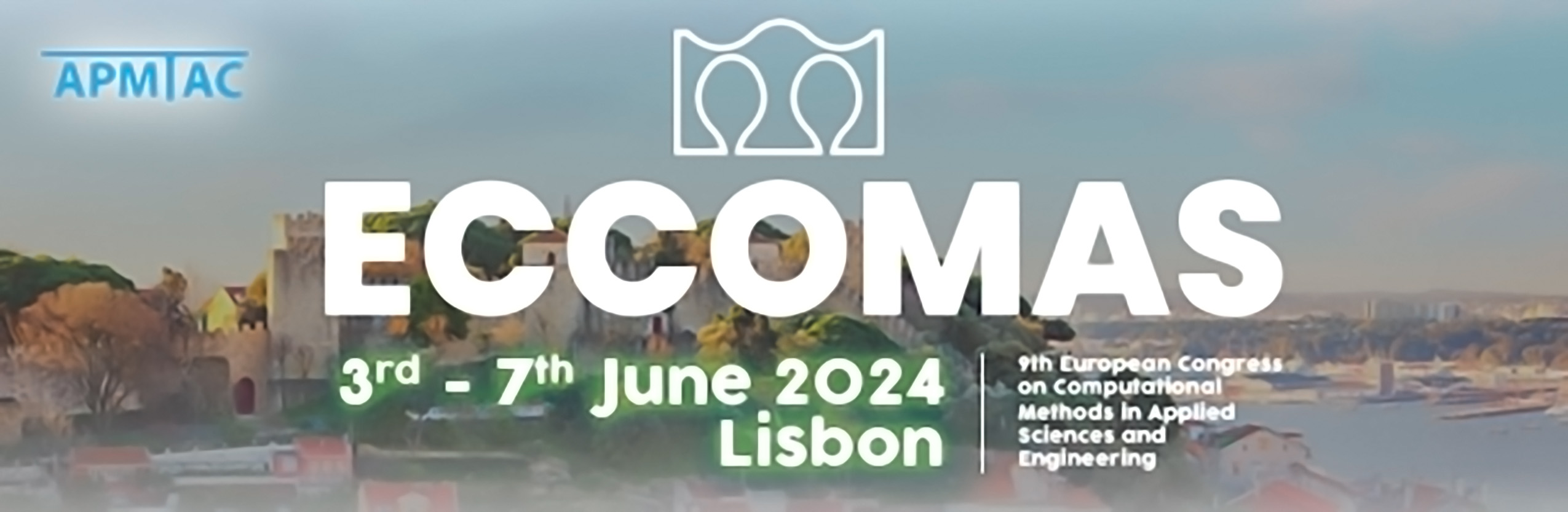
Probabilistic learning of the Purkinje network from the ECG
Please login to view abstract download link
One of the key components of the cardiac electrical system is the Purkinje network. Purkinje cells conduct excitation wave efficiently and faster than other cardiac myocytes. A functional Purkinje network is essential to sync the activations and contractions of the left and right ventricles. There are multiple ways for representing the Purkinje network with surrogate models. Here, we model it as a fractal trees, thus retaining a physiological fidelity. Regardless the representation, there is an increased interest to infer the Purkinje network from available clinical data. Considering the lack of imaging modalities for the Purkinje network, there have been attempts to recover its structure from electrical measurements such as electrocardiogram. However, the identification of the Purkinje network from the ECG is an ill-posed inverse problem for two reasons. In principle, there is an infinite number of Purkinje networks that will generate the same ECG. Second, in some diseases parts of the ventricles might be activated through the myocardium and not the Purkinje network, like in a bundle branch block. Then, it is not possible to recover the entire Purkinje network from the ECG and many trees will satisfy the measurements. In this talk, we will present a probabilistic approach to the identification of the Purkinje network from the ECG. In this sense, we aim to recover a distribution of Purkinje networks that can explain the observed measurements, rather than a single estimate. We tackle the problem by integrating a fast electrophysiology solver and Bayesian optimization for finding the optimal set of parameters. Finally, we use approximate Bayesian computation to draw samples from the posterior distribution. We provide several examples for the efficacy of our approach.