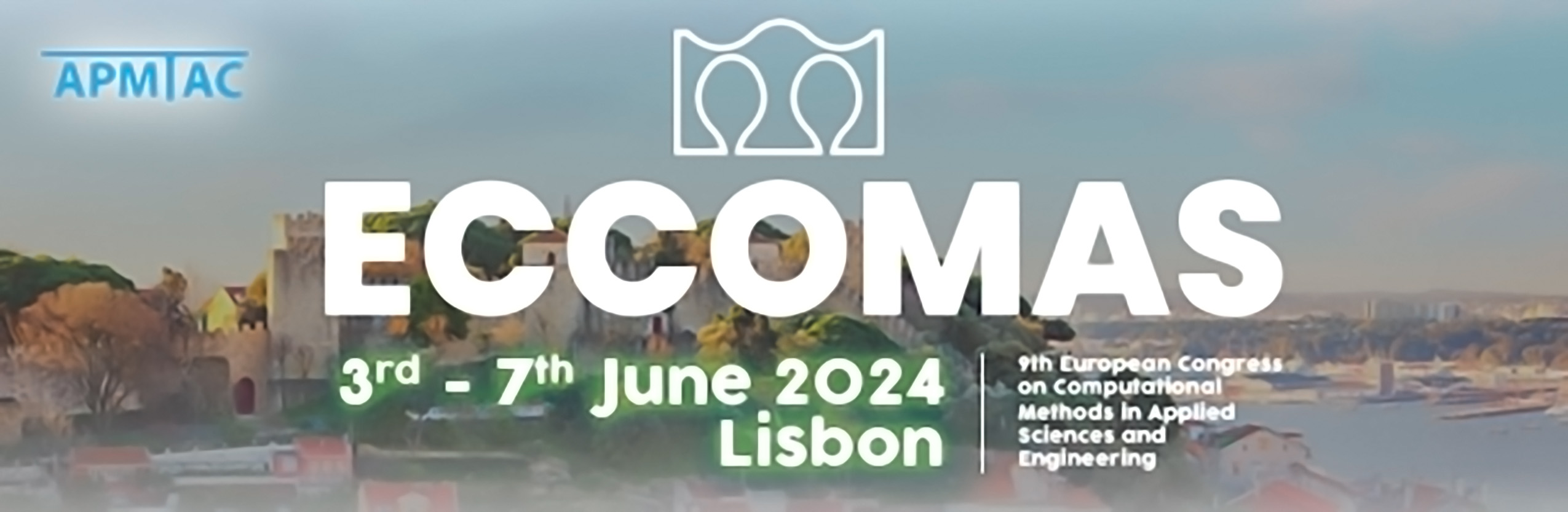
Leveraging advanced numerical calibration to filter out temperature effects on vibration-based monitoring data: application to the Mogadouro clock tower
Please login to view abstract download link
This study investigates the advantages of integrating physics knowledge to enhance traditional data-driven methods for damage detection and early warning in a monitored structure. The implemented method combines the ability to predict variations in modal properties (such as natural frequencies) under changing temperatures, using a highly reliable Finite Element (FE) model calibrated to the experimental response of the structure, with a robust anomaly detection strategy to process new monitoring data and classify it as damaged or undamaged. The relationship between temperature and modal properties, as evaluated through the FE model, is used to normalise the monitoring data. This process filters out the effects of the environmental variation, potentially magnifying the effects of damage, which are then investigated through machine learning algorithms for classification purpose. The procedure is validated by analysing a real case study, the Mogadouro clock tower in Portugal. Several scenarios of available knowledge during the training of the damage detection strategy are simulated, discussing advantages and identifying areas for future improvement.