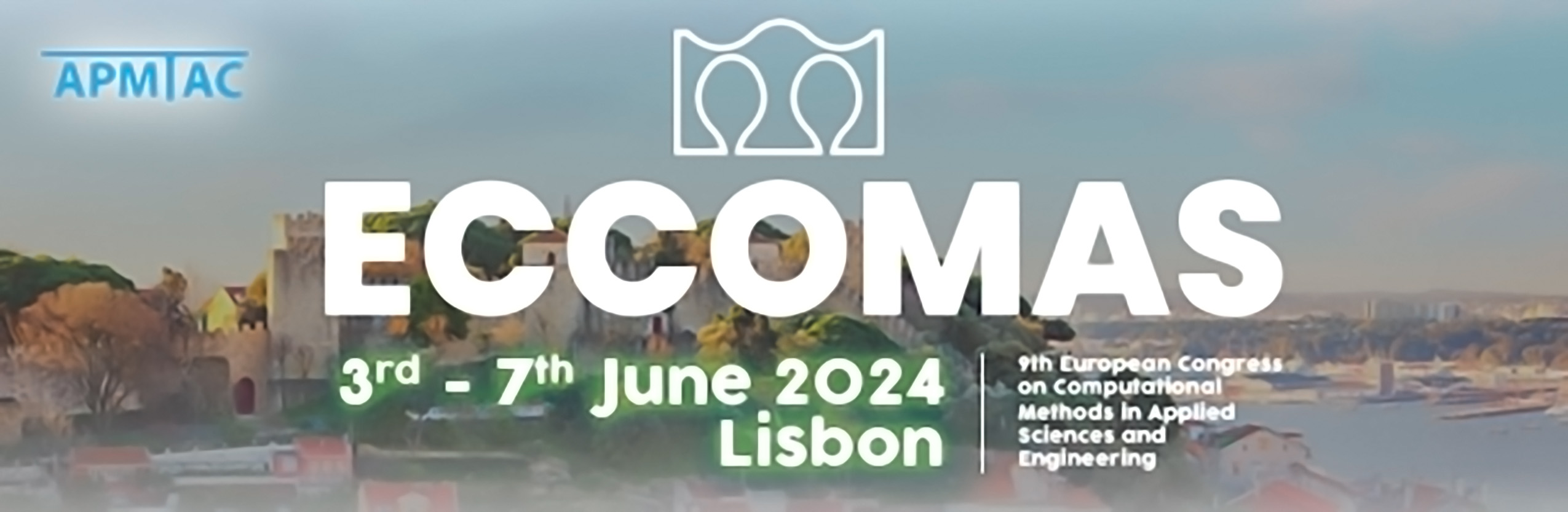
Accurate Solution of Initial and Boundary-Value Problems using Multi-level Neural Networks
Please login to view abstract download link
The solution to partial differential equations based on scientific machine learning approaches, such as the physics-informed neural networks (PINNs) method or the deep Ritz method, has shown in recent years encouraging results for several classes of initial and boundary-value problems. However, their ability to surpass, particularly in terms of accuracy, classical discretization methods remains a significant challenge. In this talk, a novel approach to reduce the numerical error for deep learning methods is proposed. The main idea consists in computing an initial approximation to the problem using a simple neural network and in estimating, in an iterative manner, a correction by solving the problems for the residual errors with new networks of increasing complexity. This sequential process allows one to significantly decrease the error in approximations of linear and nonlinear problems and achieve, in some cases, machine precision. The underlying explanation is that the method captures at each level higher frequency modes in the approximation by using a new network and a proper scaling of the residual associated with the differential equation. Numerical examples are presented to demonstrate the effectiveness of the proposed approach using PINNs.