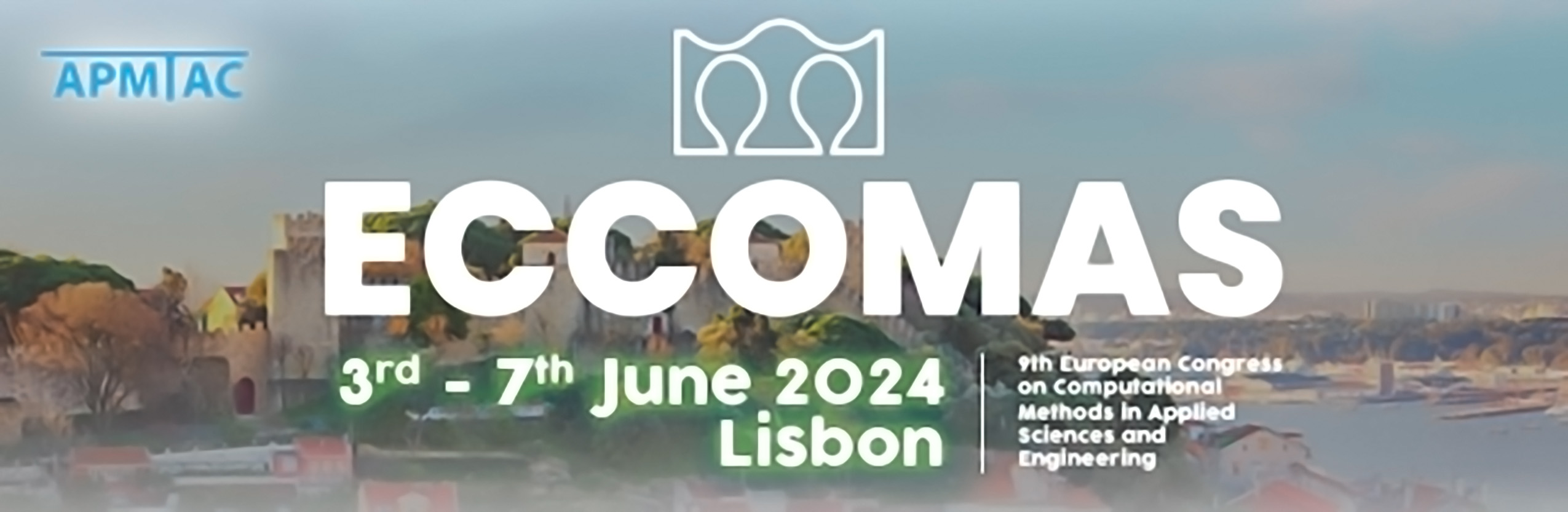
HydroGym: A Modern Reinforcement Learning Platform for Flow Control
Please login to view abstract download link
Reinforcement learning is at the heart of many modern approaches to flow control with potentially broad industrial applications such as drag reduction, mixing enhancement, and noise reduction in domains as diverse as transportation, and energy. Previously progress in the application of reinforcement learning to the flow control in these areas has been stifled by the lack of diverse, scalable reinforcement learning platforms to make approaches better comparable, and ease the barrier of entry for the development of novel approaches. In this talk we will introduce our modern reinforcement learning platform for flow control \textit{HydroGym}, a solver-independent, scalable, extensible, state-of-the-art reinforcement learning platform for rapid experimentation with non-differentiable, as well as differentiable flow control environments. We will present the performance, and rapid usability of our framework on a set of initial non-differentiable, and differentiable flow control environments before presenting a number of recent extensions further increasing the scalability, and extensibility of our framework. To this end we will present HydroGym's seamless cloud scalability using SkyPilot, its composibility with the recently introduced Koopman Reinforcement Learning, and conclude by presenting HydroGym's offline reinforcement learning user interface with its cache of reinforcement learning trajectories, and application thereof.