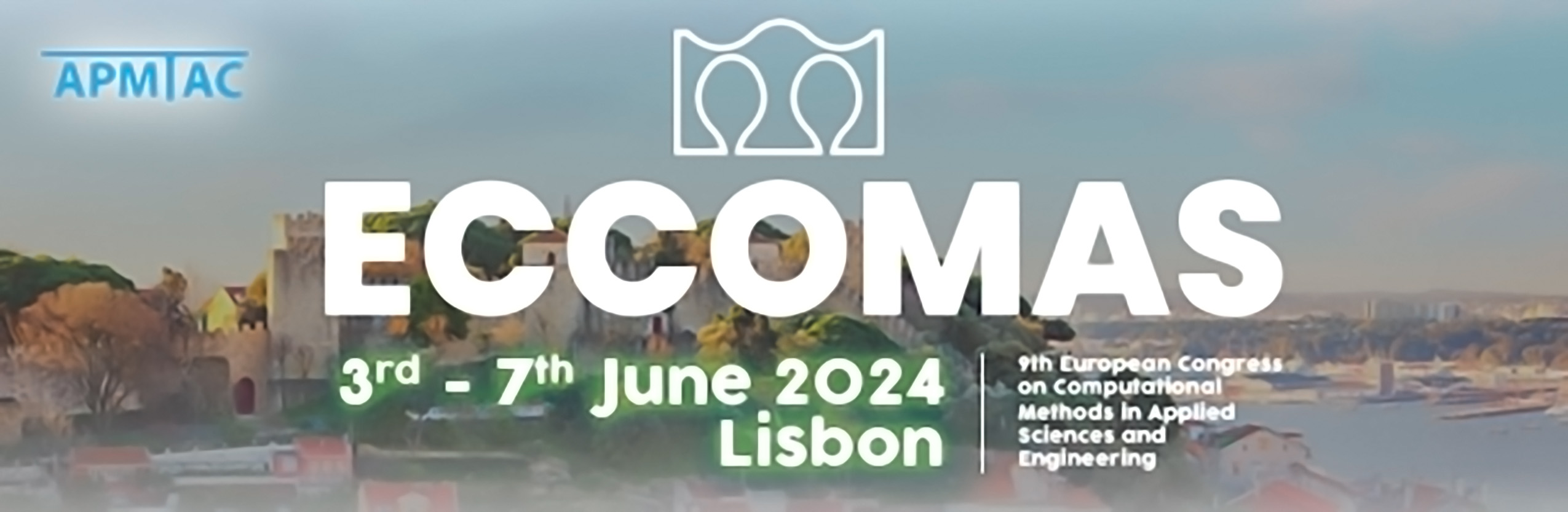
Data-informed uncertainty quantification for laser-based powder bed fusion additive manufacturing by multi-fidelity surrogate modeling
Please login to view abstract download link
We present an efficient approach to quantify the uncertainties associated with the numerical simulations of the laser-based powder bed fusion of metal processes. Our study focuses on a thermomechanical model of an Inconel 625 cantilever beam, based on the AMBench2018-01 benchmark proposed by the National Institute of Standards and Technology (NIST). The proposed approach consists of a forward uncertainty quantification (UQ) analysis of the residual strain of the cantilever beam given the uncertainty on some of the parameters of the numerical simulation, namely the powder convection coefficient and the activation temperature. The uncertainty on such parameters is modeled by a data-informed probability density function (PDF) obtained by a Bayesian inversion procedure, based on the displacement experimental data provided by NIST. To overcome the computational challenges of both the Bayesian inversion and the forward UQ analysis we employ a multi-fidelity surrogate modeling technique, specifically the multi-index stochastic collocation method. The proposed approach allows us to achieve a 33\% reduction in the uncertainties on the prediction of residual strains compared with what we would get basing the forward UQ analysis on a-priori ranges for the uncertain parameters, and in particular the mode of the PDF of such quantities (i.e., its ``most likely value'', roughly speaking) results to be in good agreement with the experimental data provided by NIST, even though only displacement data were used for the Bayesian inversion procedure.