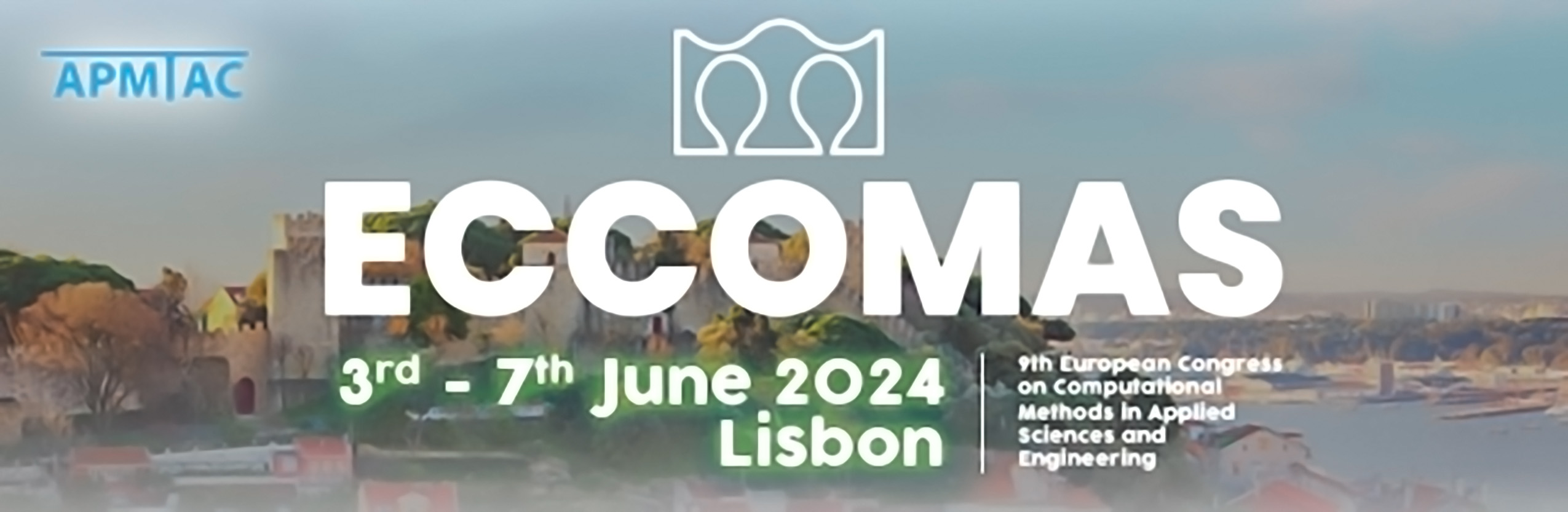
Latent Regression of Transonic Wing Pressure distributions
Please login to view abstract download link
The quest for innovation in multidisciplinary design and optimisation in aeronautics is hampered by the cost of generating new computational fluid dynamics (CFD) data. To overcome this issue, recent advances in machine learning have introduced surrogate-based methods, which combine dimensionality reduction and regression techniques to create surrogate models. These models operate in a low-dimensional space while preserving the most relevant data features, making generating CFD data less computationally demanding. Recent research has shown the promise of beta-Variational AutoEncoders (beta-VAE) in treating aerodynamic data. The beta-VAE offers nonlinear encoding/decoding capabilities, greater interpretability, latent-space disentanglement, and generalisation compared to classical autoencoders. We propose a surrogate model based on latent-space regression to predict pressure distributions on a wing given flight conditions, such as Mach number and angle of attack. A beta-VAE model maps full-order data onto a low-dimensional latent space. Subsequently, a regressor is trained to map flight condition variables onto latent space variables, completing the pipeline to provide insight into unexplored flight conditions. Ultimately, our methodology aims to facilitate a rapid, cost-effective and reliable aerodynamic shape optimisation process, reducing the need for additional simulations. The surrogate model is showcased using a database of CFD simulations of the Airbus XRF1 model, a research benchmark for a long-range wide-body aircraft, which provides a precise pressure distribution on a wing based on its flight condition.