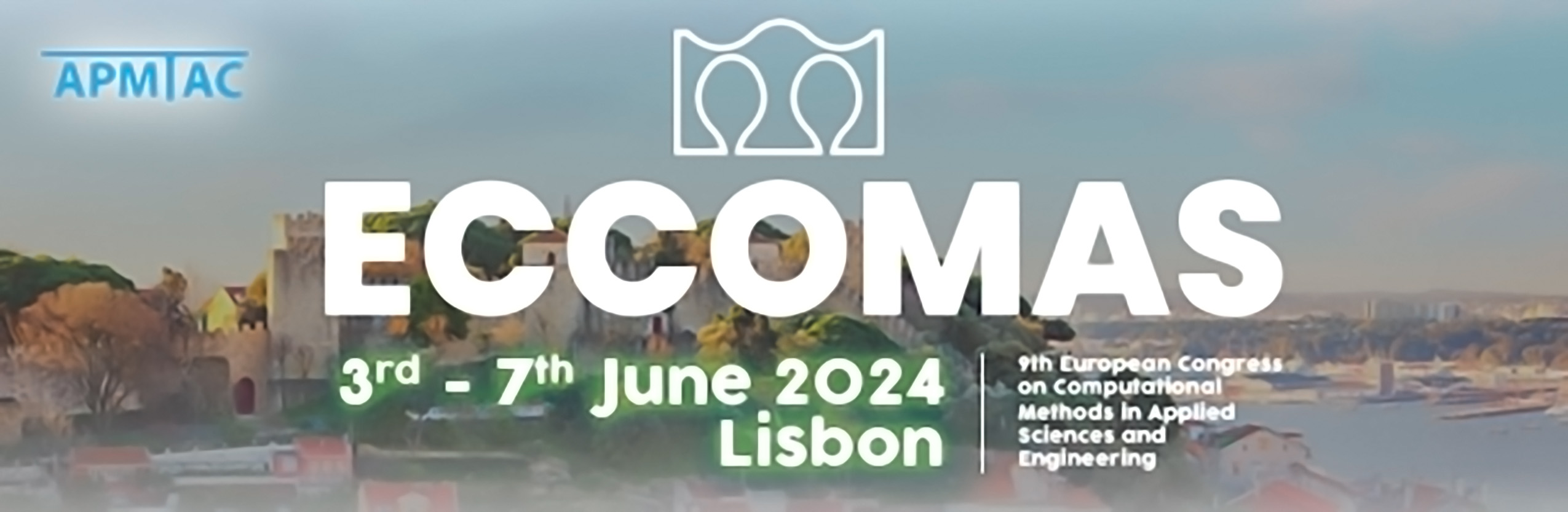
Deep-Learning Preconditioners for Mixed-Dimensional Partial Differential Equations on 3D-1D Domains
Please login to view abstract download link
Many physical problems that involve heterogeneous spatial scales, such as flow through fractured porous media, the study of fiber-reinforced materials, or the modeling of small circulation in living tissues, just to mention a few examples, can be described as mixed-dimensional problems. These are coupled partial differential equations (PDEs) defined in domains of heterogeneous dimensions that are embedded into each other. However, the algebraic structure of the resulting discretized system presents challenges in constructing efficient solution algorithms. In fact, the study of the interplay between the mathematical structure of the problem and solvers, as well as preconditioners for its discretization, is still in its infancy. The results presented in [1] for the solution of one-dimensional (1D) differential equations embedded in two-dimensional (2D), and more recently extended to the 3D-1D case in [2], have paved the way, but much more must be understood [3]. We address this challenge with a radically new approach originally proposed in [4]. We introduce a data-based technique to address the system resulting from the finite element discretisation of both 3D and 1D partial differential equations. In our method, we merge traditional iterative solvers with convolutional neural networks (CNNs) to create a preconditioned Krylov solver. The preconditioner employs the U-Net architecture, making use of CNN layers. Through our training approach, our CNN-based preconditioner is capable of generalizing across residuals coming from broad range of data that represent the action of 1D graphs within a 3D space. This work paves the way for the efficient solution of many-query problems where a mixed-dimensional problem is solved for many configurations of a microstructure shaped as a 1D/2D graph.