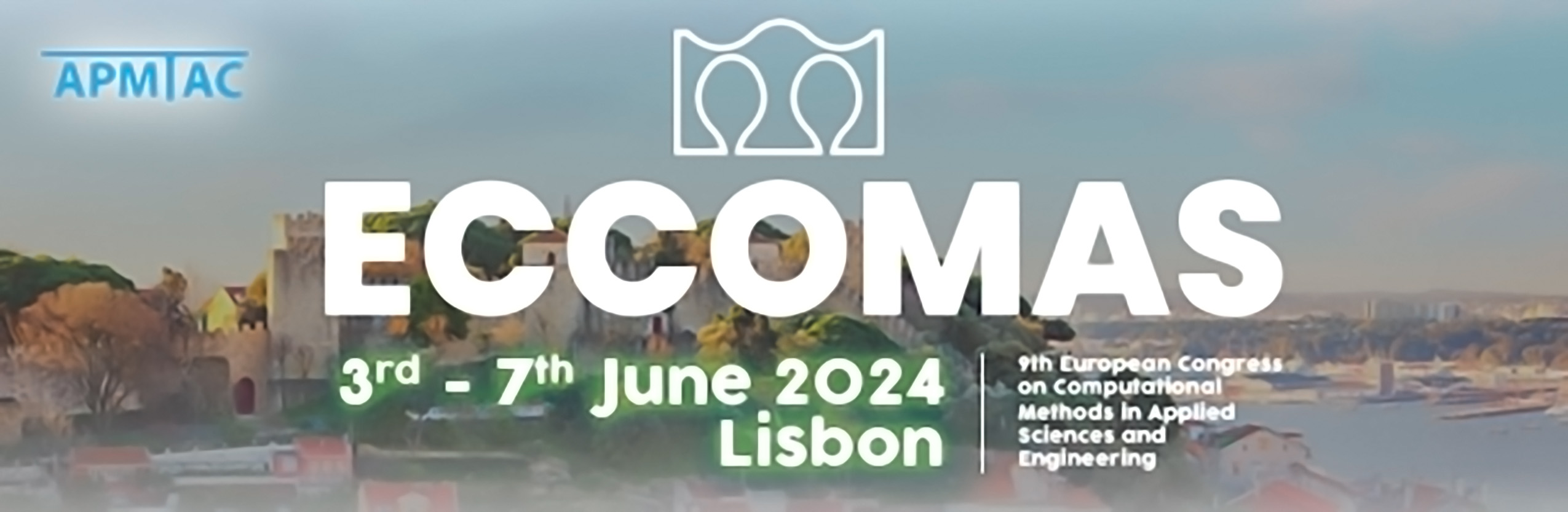
Acoustic scattering off a cylinder: surrogate modeling and inversion via physics-informed neural networks
Please login to view abstract download link
From the wide range of problems involving acoustic waves, this study delves into the scattering of acoustic waves by rigid objects, emphasizing the application of Physics-Informed Neural Networks (PINN) for forward, inverse, and surrogate modeling. While PINN exhibits a slow convergence in forward problems compared to well-established numerical methods, its distinct advantages shine in surrogate modeling and inversion, showcasing its versatility as a unified framework. Within the PINN framework, we introduce two different methodologies. First, the Boundary Condition Method: a conventional approach assuming complete knowledge of boundary conditions and governing equations. Second, the Sensor Method, where a boundary condition is replaced by data from select locations at a distant from the boundary. Both methods demonstrate PINN's strength in forward modeling and for interpolation/extrapolation in surrogate modeling. Through inverse analysis, we also establish the superior robustness of the first method over the second one.