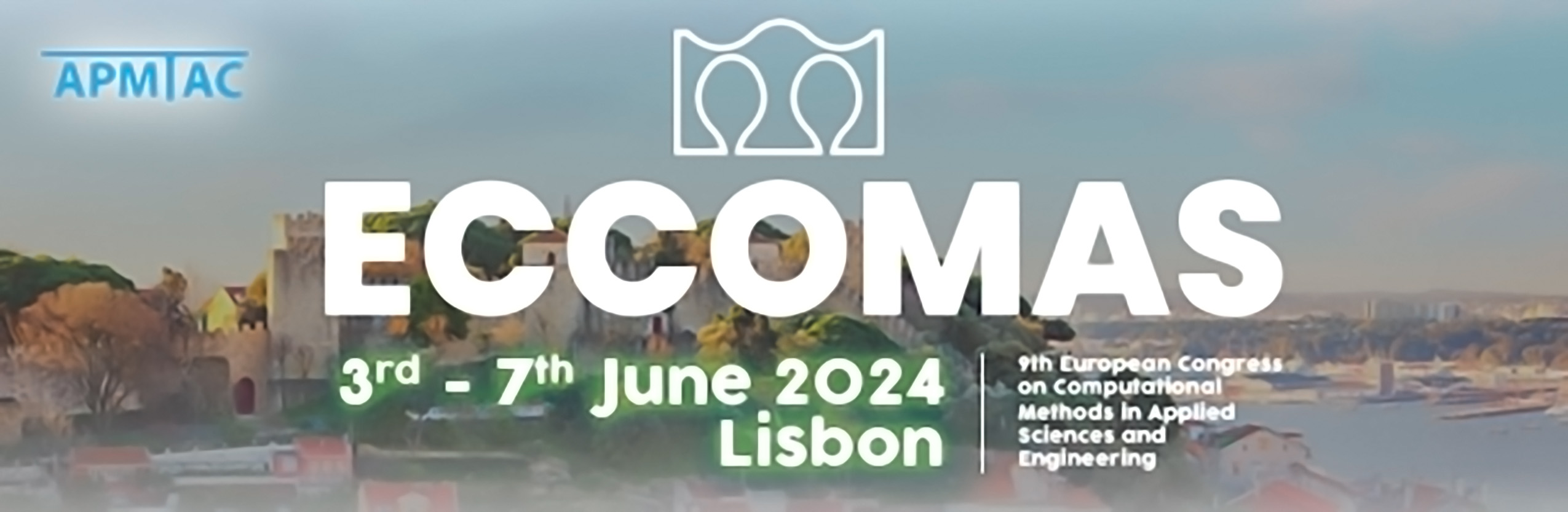
Assessing Inattend Human Elements in Critical Driving Safety Eventstiveness an
Please login to view abstract download link
Road accidents, with their potential for severe consequences, pose an ongoing global challenge. Within the multitude of factors contributing to these incidents, inattentiveness and the intricate human elements inherent in driving behaviors stand out as pivotal. As indicated by reports and studies on traffic safety, a significant share of accidents can be attributed to driver inattentiveness, encompassing activities such as texting, talking on the phone, or simply being distracted by the surrounding environment. Beyond these observable behaviors lie complex human elements, influenced by factors ranging from cognitive processes to emotional states, which significantly contribute to the occurrence and severity of critical safety events. Inattentiveness is defined as a state in which a driver's eye gaze behavior deviates from attentive driving patterns. It can be influenced by human factors and adverse weather conditions, serving as an indicator of an increased risk of inattentiveness and the potential to contribute to safety-critical events on the road. Recognition of inattentiveness occurs when the average gaze duration on the road or critical areas falls below a specified threshold. The driver's response time is crucial to the braking process of the vehicle and, therefore, has a significant impact on safety in critical situations. This study aims to pinpoint and analyze the human factors contributing to critical driving safety incidents and to measure the extent of inattentiveness among drivers during such events. The exploration encompasses the various forms inattentiveness may take and its correlation with accident severity. The motivation behind this research lies in comprehending the relationship between eye gaze patterns and various external factors, specifically human influences and adverse weather conditions, which can significantly impact driver reaction times. In understanding and evaluating inattentiveness and human factors in critical driving safety events, the proposed combination of deep learning and a bias network holds promise. Deep learning models showcase a capability to predict inattentiveness and human elements in real-time by estimating gaze angle directly from eye images and eye landmark coordinates. These models leverage advanced neural network architectures to analyze complex driving behavior patterns and are envisioned to serve as an effective early warning system, offering timely alerts when drivers exhibit signs of inattentiveness