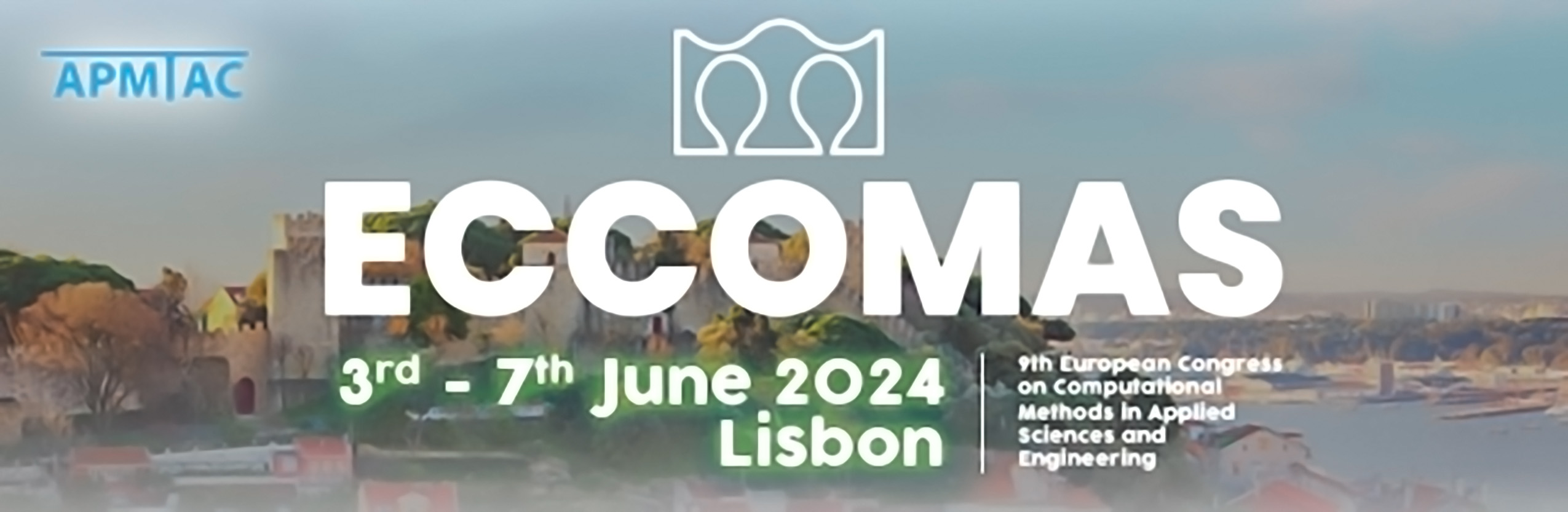
Discovering linear and nonlinear shared manifolds for enhancing multifidelity uncertainty quantification
Please login to view abstract download link
Estimating statistics of quantities of interest of computationally expensive models is challenging because the number of available evaluations is limited. Therefore multifidelity methods, which leverage low-fidelity (LF) approximations of the high-fidelity (HF) model, have been developed to reduce estimator variance without increasing the computational cost. These approaches have been shown to perform well if the correlation between HF and LF models is high, which is not not the case for many applications of practical interest in science and engineering. This occurs, in particular, in presence of dissimilar parameterization, i.e., if the models have a different number of random inputs. In this talk we show that building a shared subspace of reduced dimension between the HF and LF models is beneficial for multifidelity uncertainty propagation. In particular, we construct a shared parameterization based on low-dimensional subspaces of high variance that are obtained through either linear (adaptive basis or active subspace) or nonlinear (autoencoder) dimension reduction strategies. This methodology allows to increase the correlation between the models and yields multifidelity estimators of reduced variance. We focus, in particular, on the nonlinear approach and show how autoencoders can be employed for supervised dimensionality reduction. We consider a set of test cases to illustrate the trade-off between the cost to build the shared space and its complexity.