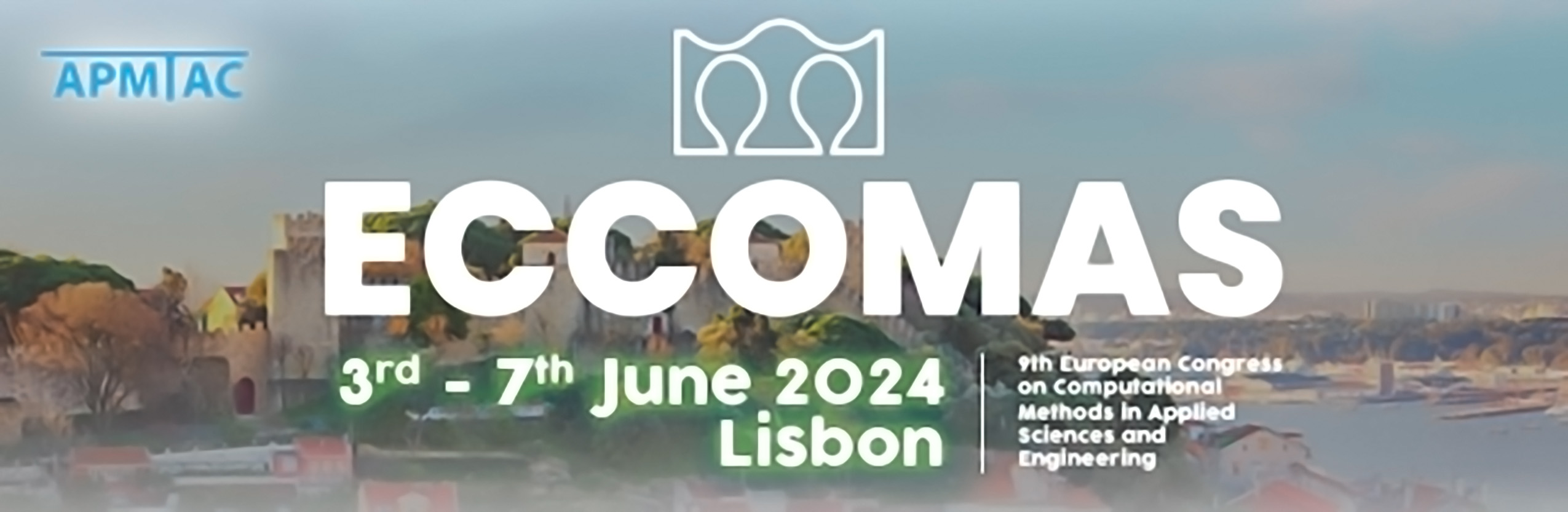
Taming the Computational Burden: PBDW for Efficient State Estimation
Please login to view abstract download link
State estimation, a crucial aspect of data assimilation, can be computationally demanding, posing challenges in its practical implementation. Conventional approaches employ variational formulations, but their computational cost often restricts their applicability. To address this issue, reduced-order models (ROMs) have been introduced, replacing the full-order model. The state estimation can be also cast as an optimal recovery approach. In this context, the Parametrized Background Data Weak (PBDW) method offers a promising strategy. Unlike variational methods, PBDW enables state estimation without parameter estimation, circumventing the complexity of nonlinear inverse problems. This work explores the application of the PBDW method to reconstruct time-dependent problems, incorporating artificial sensor measurements and sensor selection algorithms to enhance performance.