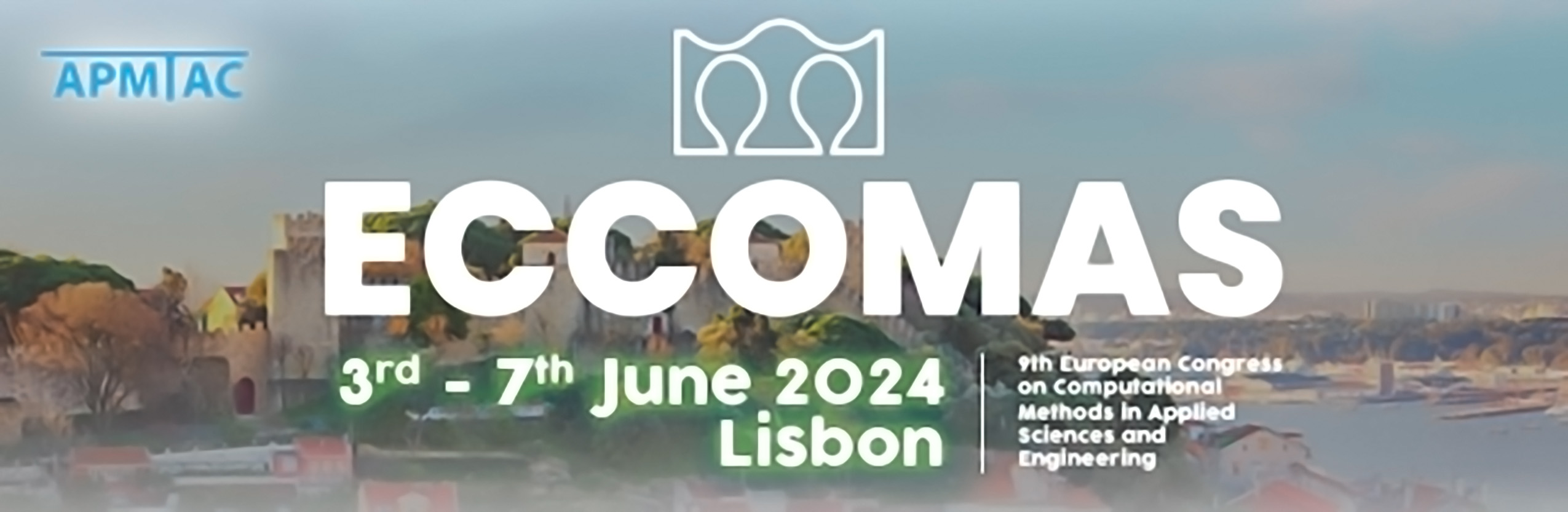
A High-Fidelity Framework Approach Enabling High-order Implicit Time Stepping For MDAO
Please login to view abstract download link
Many engineering configurations, in particular multidisciplinary scenarios and high-fidelity models, operate in complex dynamic conditions. Such configurations often can only be investigated in simplified, (pseudo-)static ways since the development of the corresponding methods and software tools for unsteady design analysis and optimizations lags behind their steady-state counterparts, and the necessary computational resources are not available. In our work, we present a framework-based approach to extend the multidisciplinary design analysis and optimization capabilities of the OpenMDAO framework towards unsteady problems. To this end, we provide new framework abstractions to build multidisciplinary instationary models via diagonally implicit Runge-Kutta (DIRK) schemes. A memory-efficient nested approach is pursued to minimize overheads associated with the multidisciplinary, implicit time-stepping: an inner problem governs the multidisciplinary coupling stage-wise. On the stage level, we take advantage of the steady-state capabilities of the underlying framework OpenMDAO, such as implicit solution algorithms and multidisciplinary gradient accumulation. The inner problem is run by an outer component handling the time integration and updating its inputs when cycling over the stages and time steps, respectively. We demonstrate and verify the framework extension for unsteady problems for different test cases, including a set of ordinary differential equations and selected CFD-coupled problems. In the latter case, we have integrated the computational fluid dynamics (CFD) software CODA, developed as part of a collaboration between the French Aerospace Lab ONERA, the German Aerospace Center (DLR), Airbus, and their European research partners. To support the use of preconditioned Newton-Krylov schemes on the multidisciplinary level of the time stages, the CFD solver integration offers API methods, e.g. to trigger disciplinary block inversions, residual computations and the calculation of the corresponding derivatives by means of algorithmic differentiation.