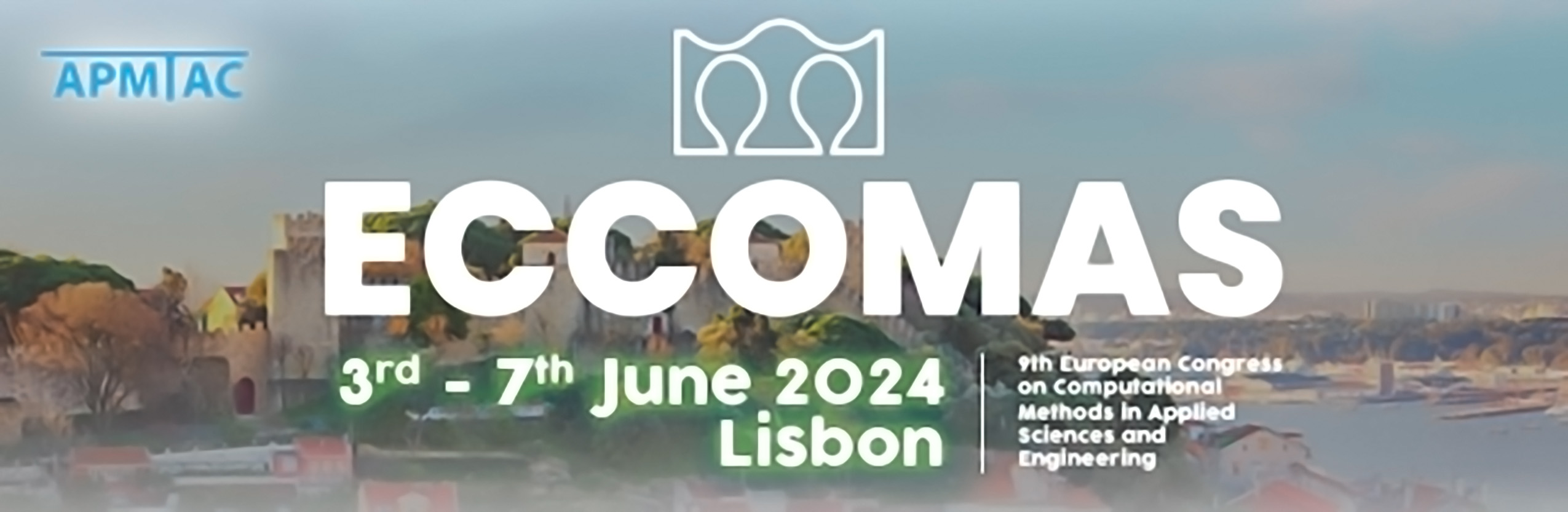
Robust design optimization using adaptive Kriging and extreme value distributions.
Please login to view abstract download link
This work focuses on Robust design optimization under interval epistemic uncertainty relevant in cases of extremely limited prior information. Within this framework robustness can be seen as the width of the output interval given the input interval. Robust design optimization problems suffer limitations associated with the computation burden of solving the two nested optimization problems especially when associated with numerical models. This work makes use of the adaptive Kriging framework to alleviate the computation burden. The principle is to take advantage of the stochastic nature of the Kriging estimation as an indicator of its localized accuracy. In the context of robust design optimization, the quantity of interest is the width of the output interval for a given set of design parameters. While the Kriging counterpart of this quantity is a random variable its distribution cannot be directly extracted. Existing methods proposed to rely e.g. on confidence interval estimations[1] or implementing two nested EGO algorithms[2,3]. This work explores an alternative approach based on the extreme values (EV) distribution associated with the Gaussian outputs of the surrogate. The idea is to extract an indicator distribution of the robustness based on the difference between two EV distributions. This indicator only requires the resolution of two (inner-)optimization problems, which represents a significant improvement upon existing methods. In turn, this indicator distribution yields some bias with respect to the true unknown distribution. Several ways of defining the indicator distribution have been explored and compared and early results look promising on benchmark examples. [1] Conradus van Mierlo, Augustin Persoons, Matthias G.R. Faes, David Moens, Robust design optimisation under lack-of-knowledge uncertainty, Comput. Struct. 275 (2023) 106910–106910. https://doi.org/10.1016/j.compstruc.2022.106910. [2] D.R. Jones, M. Schonlau, Efficient Global Optimization of Expensive Black-Box Functions, J. Glob. Optim. (1998) 38. [3] J. Marzat, E. Walter, H. Piet-Lahanier, A new expected-improvement algorithm for continuous minimax optimization, J. Glob. Optim. 64 (2016) 785–802. https://doi.org/10.1007/s10898-015-0344-x.