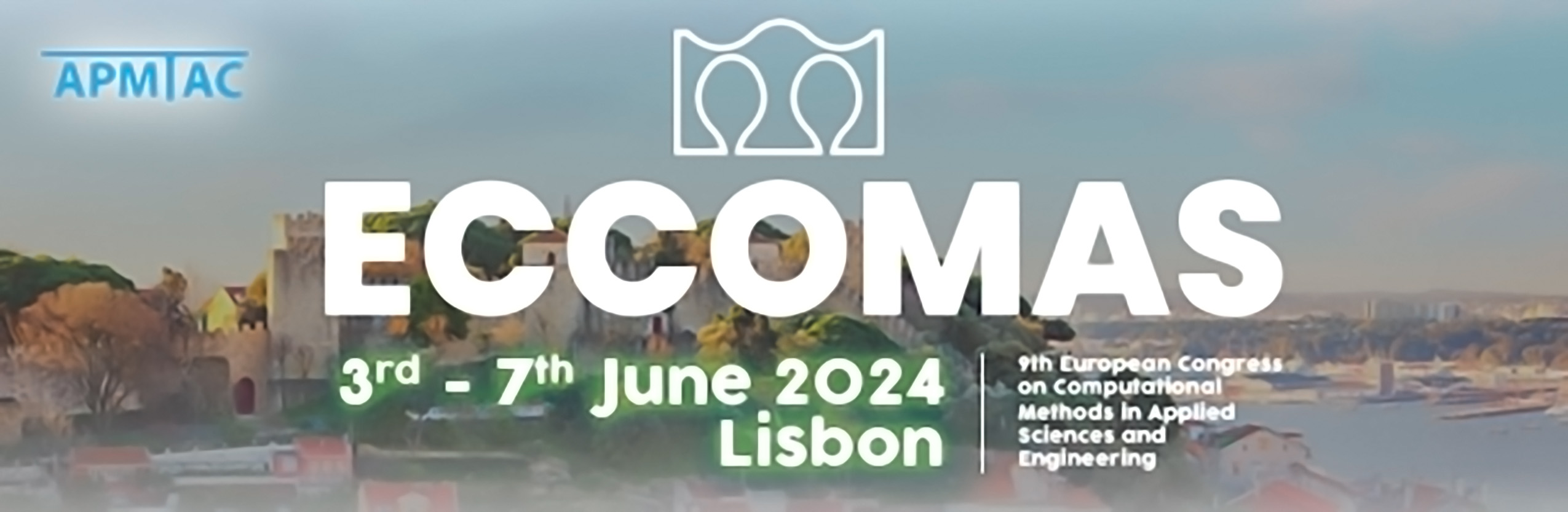
Real-time anomaly detection of spatial processes via conformal prediction and functional data analysis
Please login to view abstract download link
Temporally variant data observed on two-dimensional domains arise naturally across several disciplines, prompting a growing demand for dedicated analysis techniques. Functional data analysis [1] proves inherently suitable for representing and modelling this kind of data, offering a rigorous mathematical framework capable of preserving the spatially continuous nature of these data. Within this framework, discrete-time evolving surfaces can be effectively modelled as functional time series (i.e., a sequence of temporally dependent random variables valued in functional space) of random real-valued functions defined on a two-dimensional domain. Building upon this approach, an anomaly detection method for handling such data is here developed. The central focus revolves around conformal prediction, a versatile nonparametric paradigm used to quantify prediction uncertainty [2] and in detail on recent variations of conformal prediction for functional time series [3]. The proposal hinges on a probabilistic forecasting scheme for two-dimensional functional time series, extending a functional autoregressive process of order one to this context and incorporating conformal prediction bands for functional data recently introduced in [4]. This methodology allows the real-time construction of a spatially varying prediction range for each point of the spatial domain ensuring global control of the coverage probability. The latter represents the probability that the newly observed surface is contained within the prediction range for each point in the domain. An anomaly is identified every time the observed surface deviates from the prediction range at a particular point in the domain. This approach inherently guarantees the exact control of the probability of encountering one or more false warnings across the spatial domain, offering a viable solution for real-time monitoring of high-resolution spatial data. Finally, to illustrate its applicability, the proposed anomaly detection procedure is applied to a dataset collecting daily observations of sea level anomalies of the Black Sea and to a dataset collecting weekly interferometric measures of land elevation speed in the Phlegraean Fields volcanic area (Italy).